Mastering Transfer Learning: Techniques and Ethical Frontiers
May 15, 2024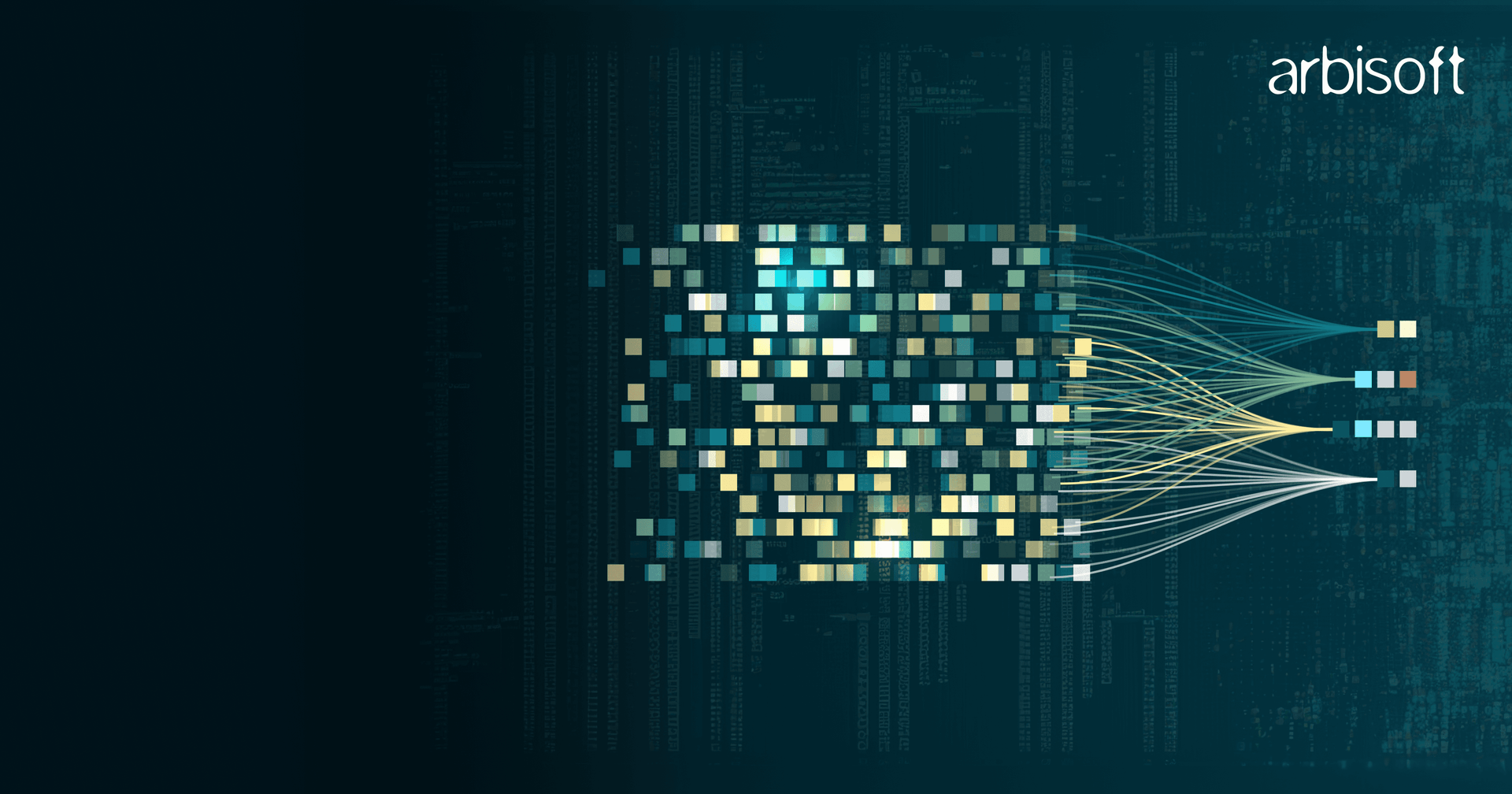
Transfer learning is a crucial aspect of AI, serving as a key element that enables limitless creativity. In this exploration, we won't just skim the surface; instead, we'll delve into specific techniques, uncover real-world applications, and confront the ethical challenges that define this dynamic field.
This blog builds on our explorations of GenAI fundamentals and transfer learning, offering advanced techniques and ethical discussions.
So let’s dive in.
Navigating Transfer Learning Techniques
In the vast horizon of artificial intelligence, transfer learning has emerged as a powerful navigation tool, allowing practitioners to tackle the complexities of model adaptation. It's a journey beyond mere model training. It is a process where pre-existing knowledge becomes a compass guiding models to new horizons.
Let's embark on an exploration of techniques—fine-tuning, feature extraction, and domain adaptation—that serve as waypoints in this transformative journey. We'll unravel the intricacies of these techniques, equipping you to navigate the seas of transfer learning.
Fine-Tuning: A Symphony of Precision
Much like adjusting the focus of a camera lens, fine-tuning allows practitioners to harmonize pre-trained models for specific tasks. Refining hyperparameters is undoubtedly crucial in the process of fine-tuning a model, yet it's just one part of the intricate procedure. Equally vital is the retraining of the model's parameters, either partially or entirely, leveraging a dataset distinct from the one used for its initial training. This multifaceted approach is exemplified in a recent study by Stanford researchers, where they illustrated the potency of fine-tuning a BERT model for sentiment analysis. Their findings underscore the intricate interplay between achieving model generalization and tailoring it to the nuances of a specific task.
Feature Extraction: Unveiling Hidden Melodies
Feature extraction is where the latent melodies of transfer learning come to life. A notable example, demonstrated by Donahue et al. (2014), involved transferring features from a Convolutional Neural Network (CNN) pre-trained on object recognition. The original CNN was trained on a vast dataset of diverse images, encompassing various objects and scenes. When fine-tuned on a different dataset, perhaps one focusing on medical imaging, the CNN adapted its learned features to better suit the new domain. This adaptation elevated performance across a diverse array of image-related tasks, capturing abstract patterns and details that empower models to compose nuanced visual and linguistic masterpieces.
Domain Adaptation: Bridging Worlds
In the realm of domain adaptation, real-world applications abound. Researchers at Google (Sun et al., 2016) employed domain adaptation techniques to improve the accuracy of speech recognition models across various environments, including but not limited to noisy environments, accented speech, and diverse acoustic conditions. It's the art of making your model a versatile linguist and a visual virtuoso, seamlessly navigating the nuances of diverse datasets.
Real-World Marvels: Transfer Learning in Action
Step beyond the theoretical and witness the tangible impact of transfer learning across diverse industries. It's not just about models in isolation; it's about how these technological wonders shape and revolutionize our everyday experiences.
a. Healthcare: Diagnosing Beyond Boundaries
Transfer learning isn't just a hypothetical concept; it's a real-world technology transforming healthcare. The groundbreaking research from the Journal of the American Medical Association (Rajpurkar et al., 2017) is a beacon in this transformative journey. It illuminates how transfer learning, leveraging the knowledge acquired from ImageNet pre-trained models, acts as a catalyst in the early diagnosis of diseases through medical imaging. This isn't merely about recognizing images - it's about bridging the gap between the broad strokes of general image recognition and the precision required for specialized medical diagnostics.
Similarly, in COVID-19 detection from chest X-rays, researchers have successfully employed features extracted from pre-trained models, demonstrating the versatility and effectiveness of domain adaptation methods in addressing critical challenges across different domains.
b. Finance: Predicting Financial Crescendos
In the dynamic realm of finance, transfer learning proves its mettle in predicting market trends. A study by Li et al. (2014), - a testament to the fusion of quantitative analysis and machine learning prowess.
Picture this: a model, initially pre-trained for general tasks, undergoes a metamorphosis through feature extraction and fine-tuning. Its predictive power is elevated to the point where it becomes a reliable indicator in the intricate flow of financial markets.
This isn't just about numbers and algorithms; it's about understanding the nuances of market dynamics, discerning patterns, and forecasting trends that elude conventional methods. The collaboration between data science and financial expertise resulted in a predictive tool that not only adapted to changing market conditions but anticipates them. It aims to provide invaluable insights for investors, analysts, and financial decision-makers.
Ethical Frontiers: Beyond the Binary
Finally, let's tackle the elephant in the room: Ethics. As we empower models to generate content, we tread a fine line. Bias in data can perpetuate stereotypes, and AI-generated content can inadvertently spread misinformation. Striking a balance between innovation and responsibility is the code we must write.
Deepfakes: The Dark Side of Creativity
In our relentless pursuit of pushing the boundaries of AI creativity, we find ourselves grappling with the shadows it casts. Deep within this technological frontier of Generative AI lies the ethical quagmire of deepfakes, a realm where the seemingly boundless potential of transfer learning transforms into a double-edged sword.
Deepfakes involve the synthesis of hyper-realistic digital content, often using artificial intelligence to manipulate or fabricate videos, audio, images, and text. This also includes generating fake news, hate speech, and other deceptive text-based content. While these advancements showcase the remarkable capabilities of AI, they simultaneously pose profound ethical challenges. Issues of misinformation, privacy invasion, and the potential for malicious use underscore the complex landscape of deepfakes.
As we navigate this uncharted territory, it becomes crucial to strike a delicate balance between fostering innovation and addressing the ethical considerations associated with the darker aspects of creative technology. The exploration of deepfakes prompts reflection on the responsible deployment of AI, emphasizing the need for vigilance and ethical frameworks to guide our journey into the ever-evolving landscape of artificial intelligence.
Striking the Balance: Precision with Personality
As we traverse the technical landscapes, let's not forget the human touch. Injecting a dash of personality into the discourse makes the journey more engaging. Consider this not just a technical odyssey but a creative exploration, where the formalities of code meet the whimsy of imagination.
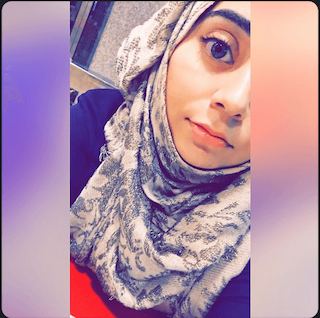
Hijab-e-Fatima
Technical Content Writer