The Resource Crisis
By 2022, training a single large language model could consume more energy than 100 American households use in a year. This crisis echoed an ancient pattern: every complex system eventually faces the limits of its resources. Just as ancient civilizations had to optimize their resource usage or collapse, AI faced its own moment of reckoning.
The Fundamental Trade-off
Model Capability vs Resource Usage:
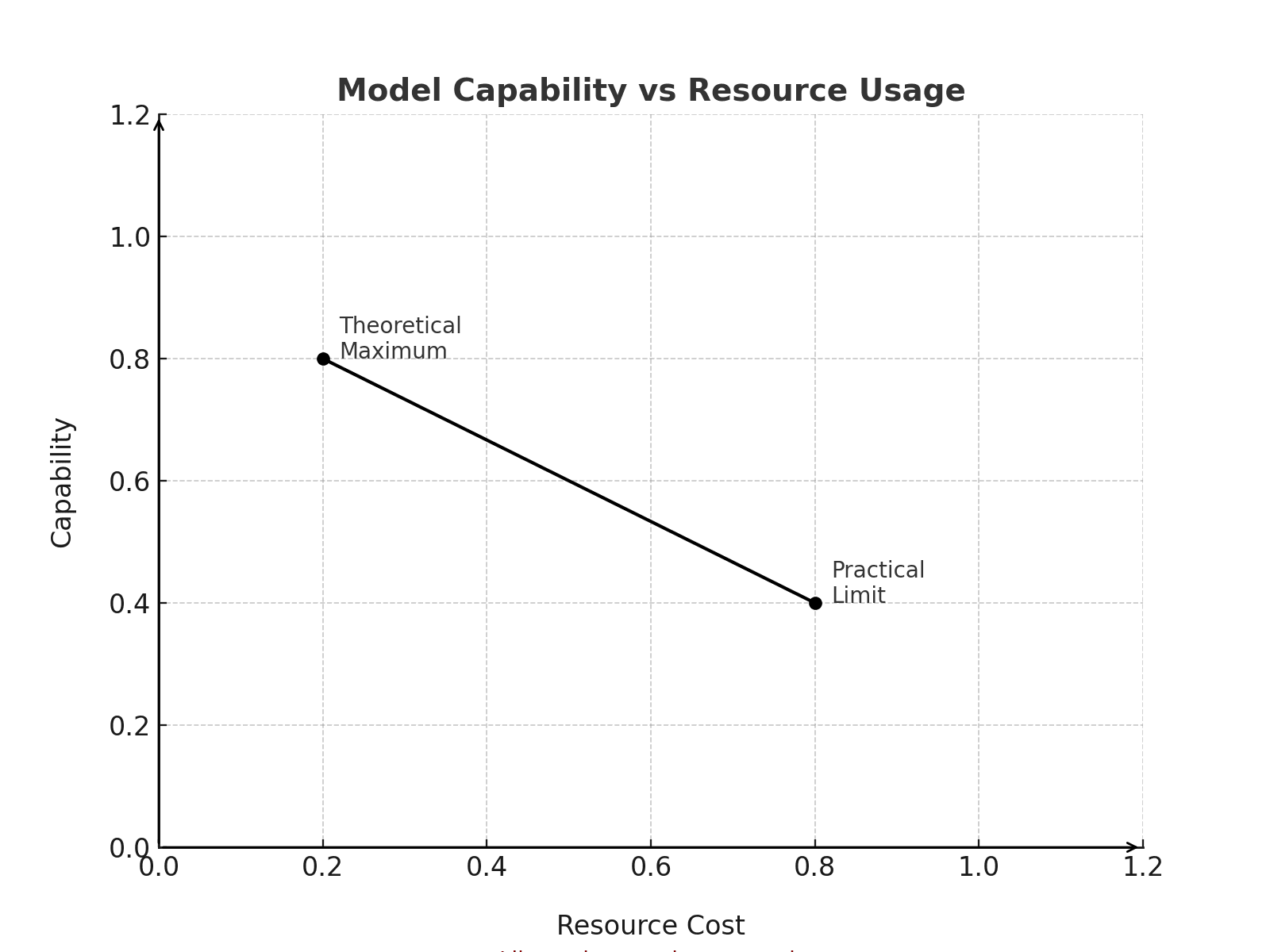
Like a city growing upward: at some point,
the cost of adding each new floor outweighs its benefits.
The Mathematics of Less is More
Pruning: The Art of Selective Forgetting
Nature had already solved this problem. During adolescence, human brains undergo synaptic pruning, removing unused connections to optimize neural pathways. AI researchers took inspiration from this biological process:
Magnitude-based Pruning:
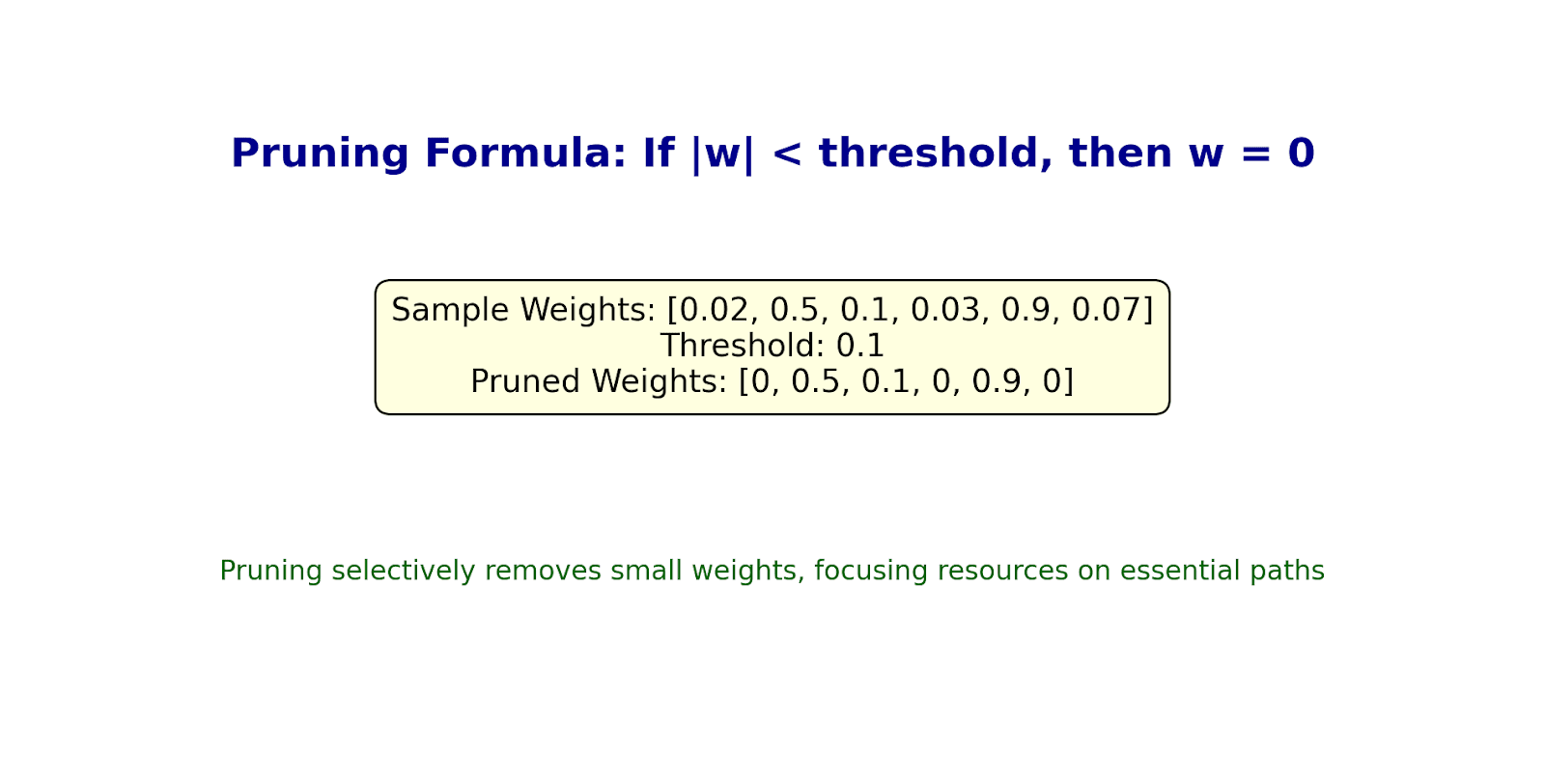
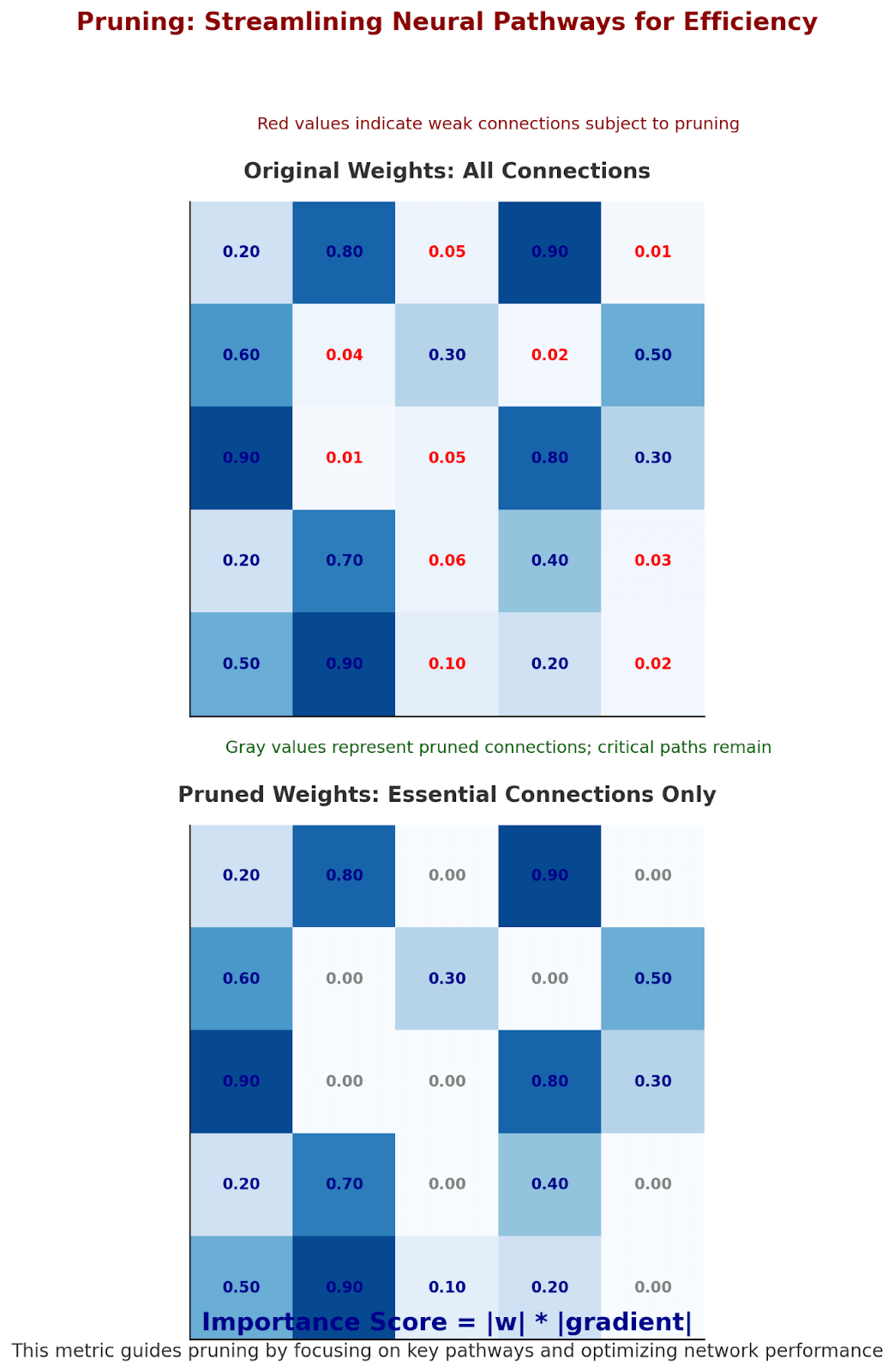
Like a gardener pruning a tree:
- Small, weak branches are removed
- Strong, essential pathways remain
- The tree grows more efficiently
But the real insight came in understanding which connections to keep. The mathematics tells a beautiful story:
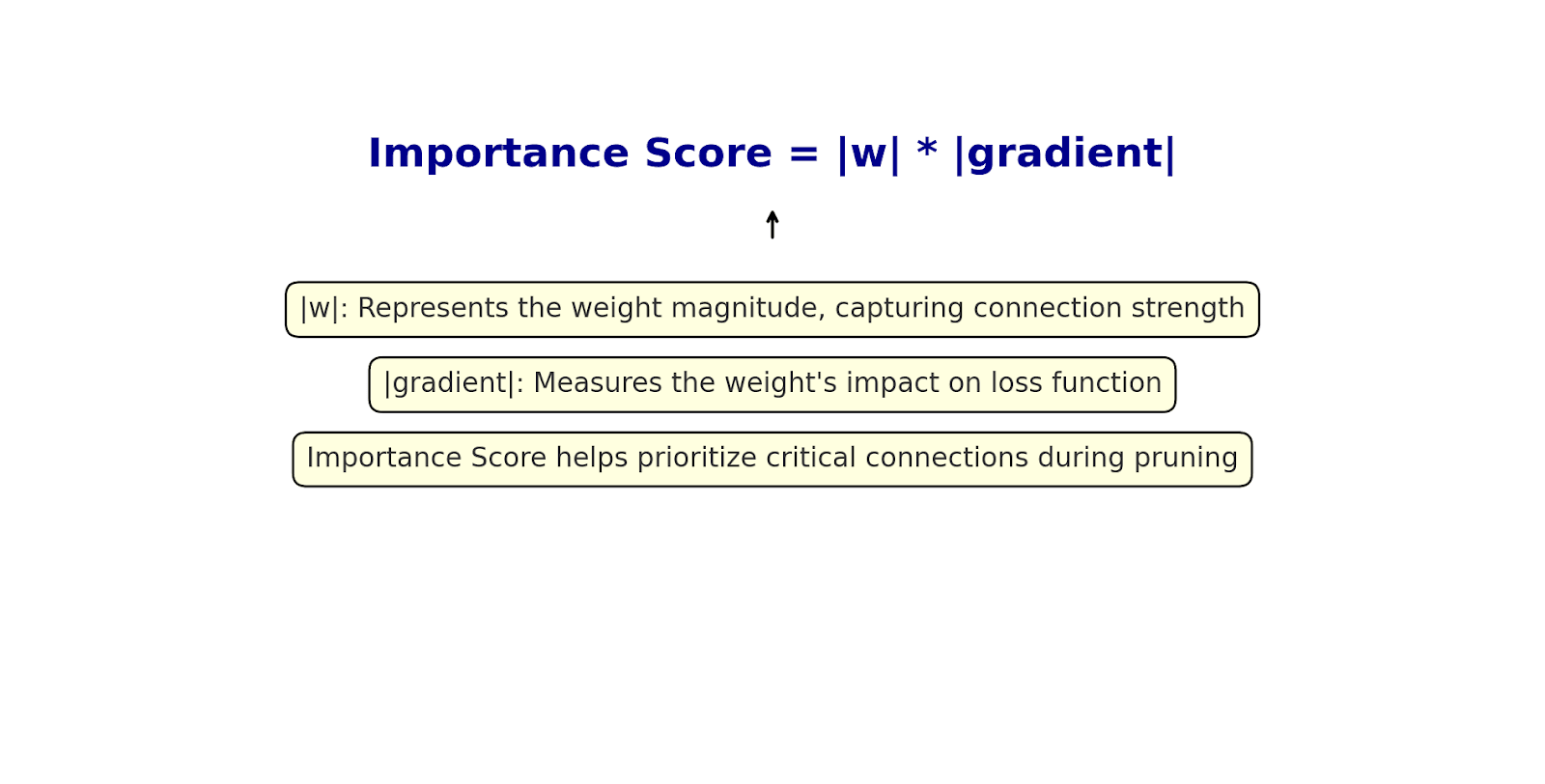
This mirrors how our brain strengthens frequently used neural pathways while letting unused ones fade.
Quantization: The Poetry of Precision
Traditional neural networks used 32-bit floating-point numbers for weights, like using a dictionary to spell "cat." Quantization showed we could use much less:
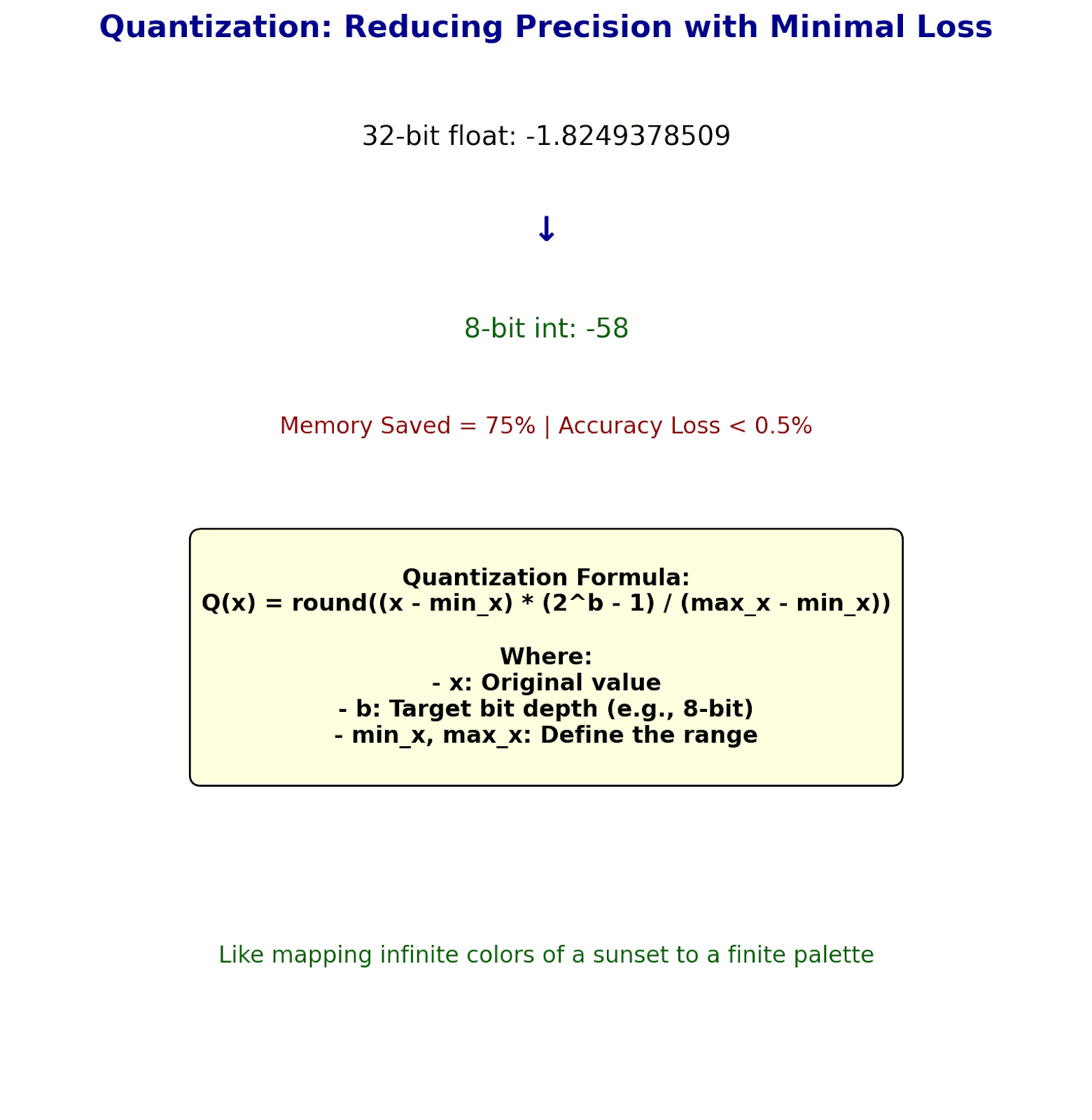
Like human language evolution: We don't need infinite precision to communicate meaning.
The mathematics of quantization tells a story of elegant approximation:
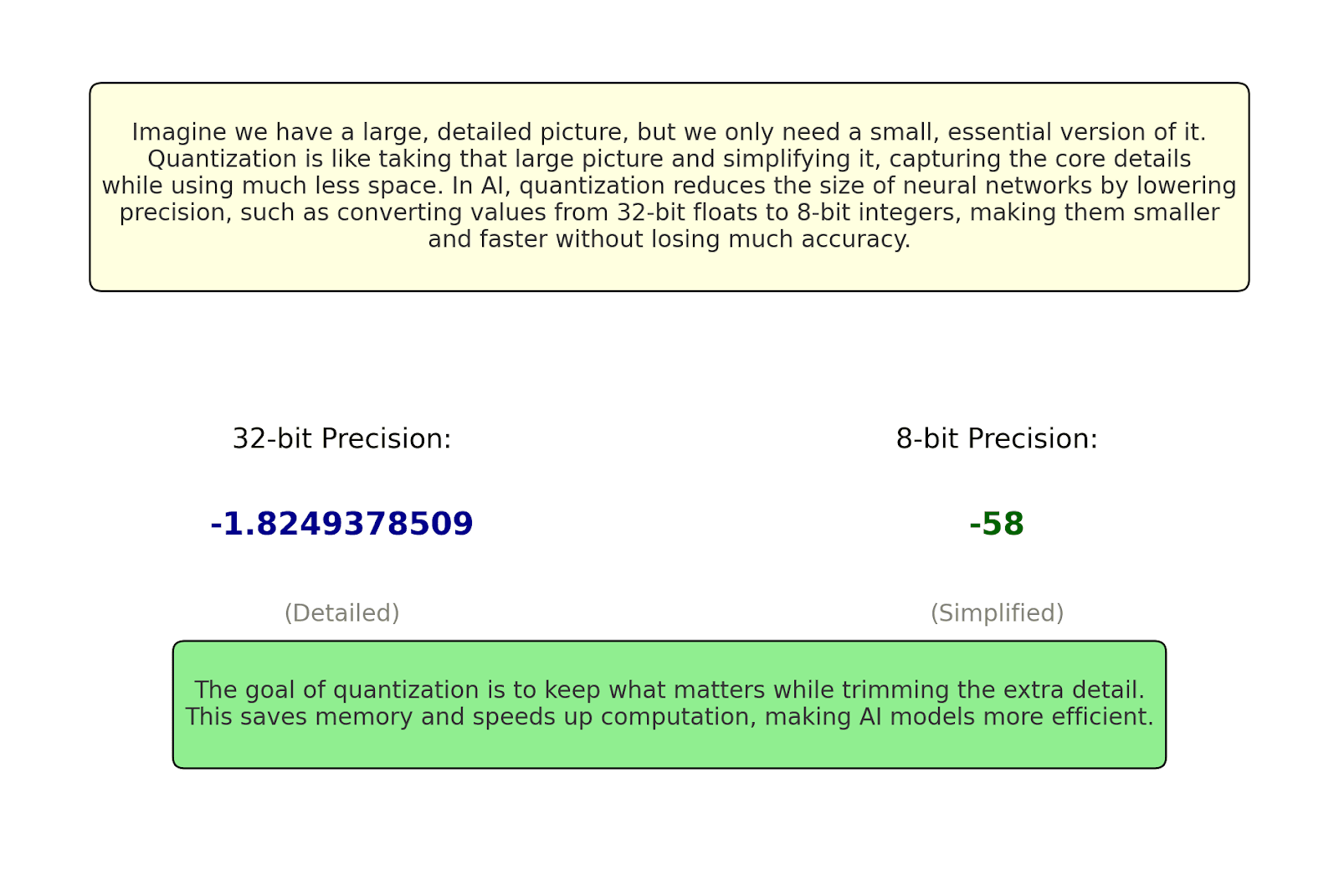
This is like mapping the infinite colors of a sunset into a finite but beautiful palette.
Knowledge Distillation: Teaching the Next Generation
Perhaps the most profound compression technique mirrors how human knowledge passes through generations. A larger "teacher" model guides a smaller "student":
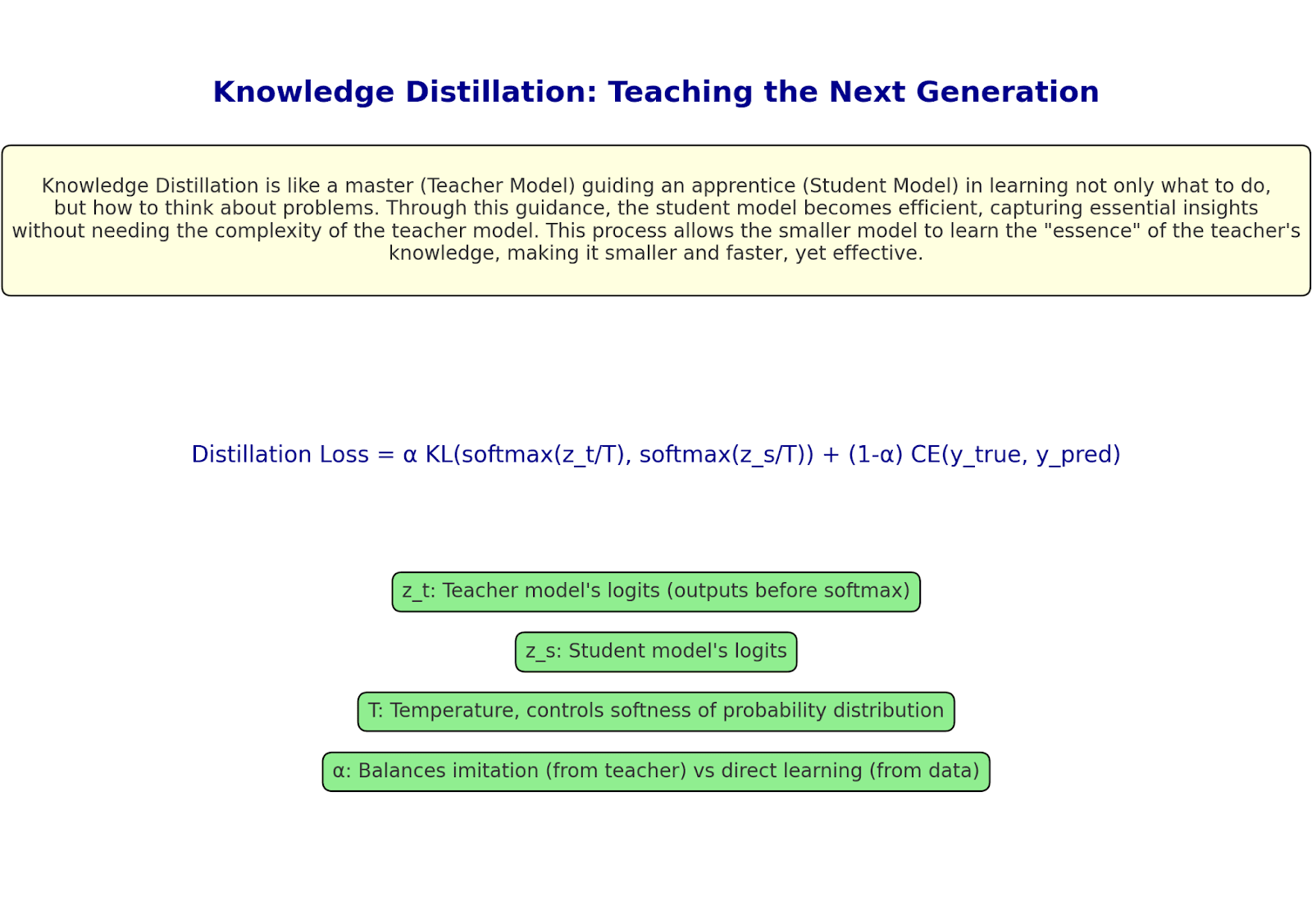
Like a master teaching an apprentice:
- The student learns not just the what, but the how
- Subtle patterns transfer through example
- The apprentice becomes efficient through guidance
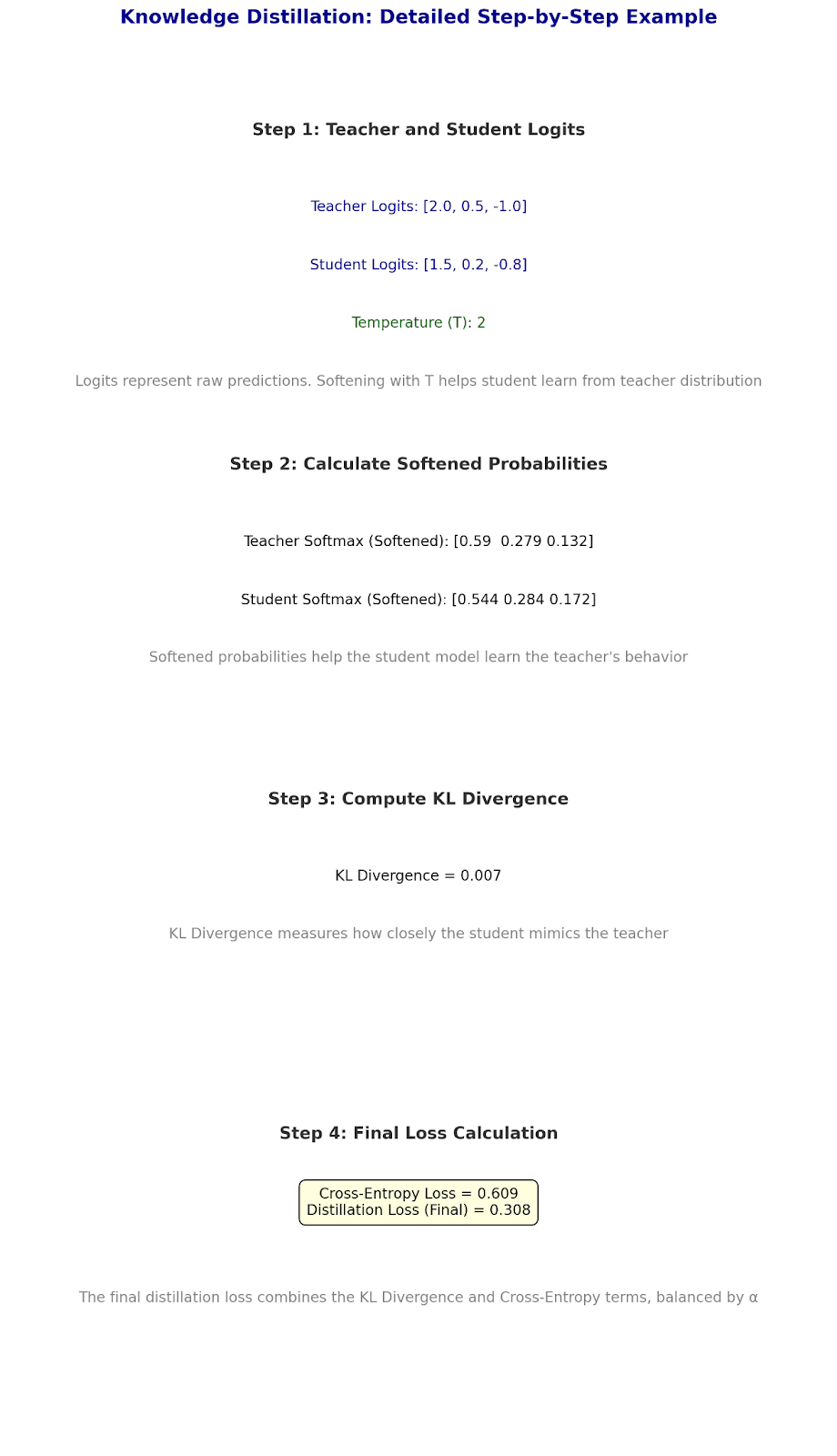
The Modern Compression Symphony
Low-Rank Approximation: Finding Hidden Patterns
Consider how our brain compresses memories, we don't store every detail, but rather the essential patterns. Low-rank approximation does the same:
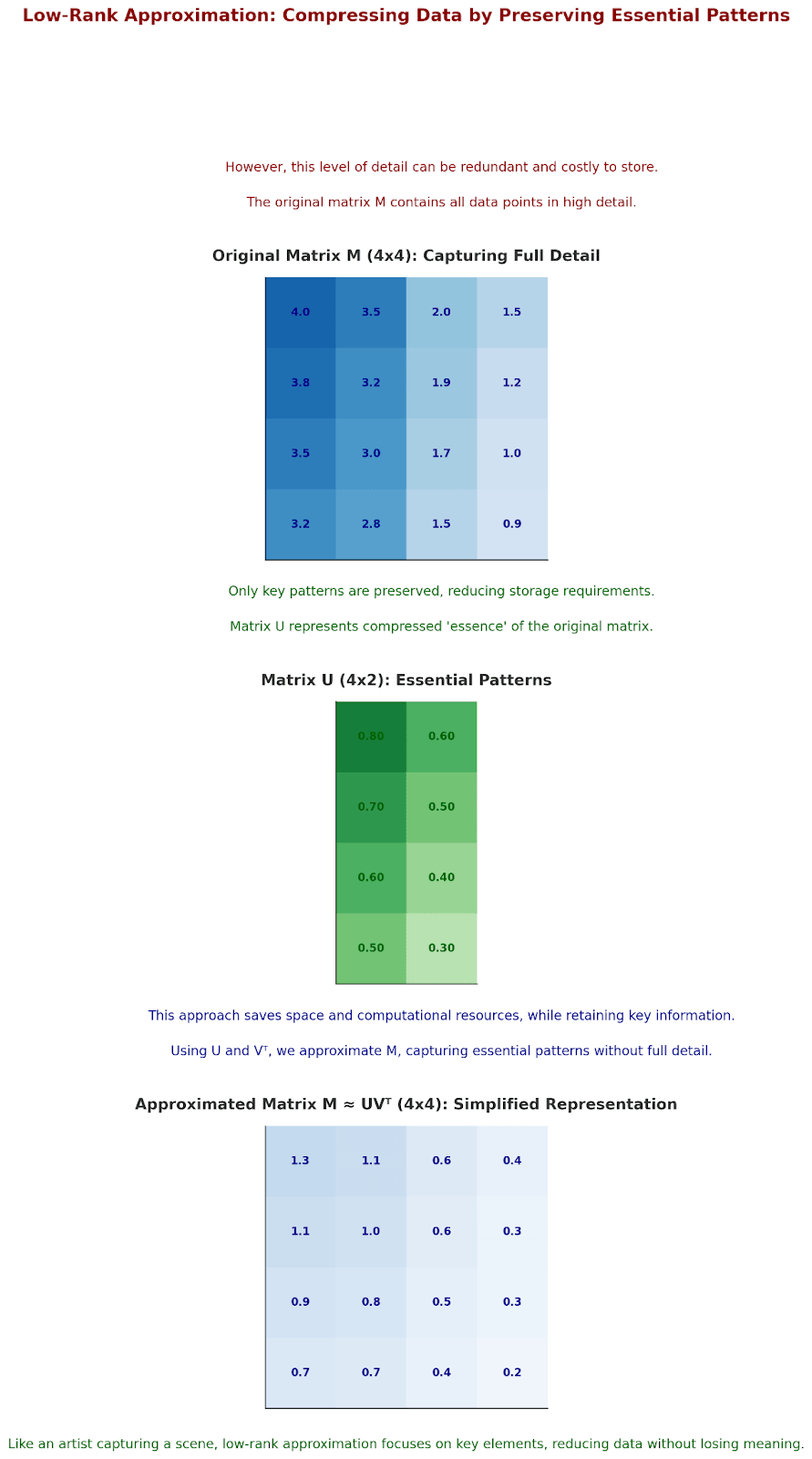
Like an artist capturing a landscape: They don't paint every leaf, but rather the essential patterns that create the scene.
The Emergence of Adaptive Computation
The latest revolution comes in models that adapt their complexity to the task:
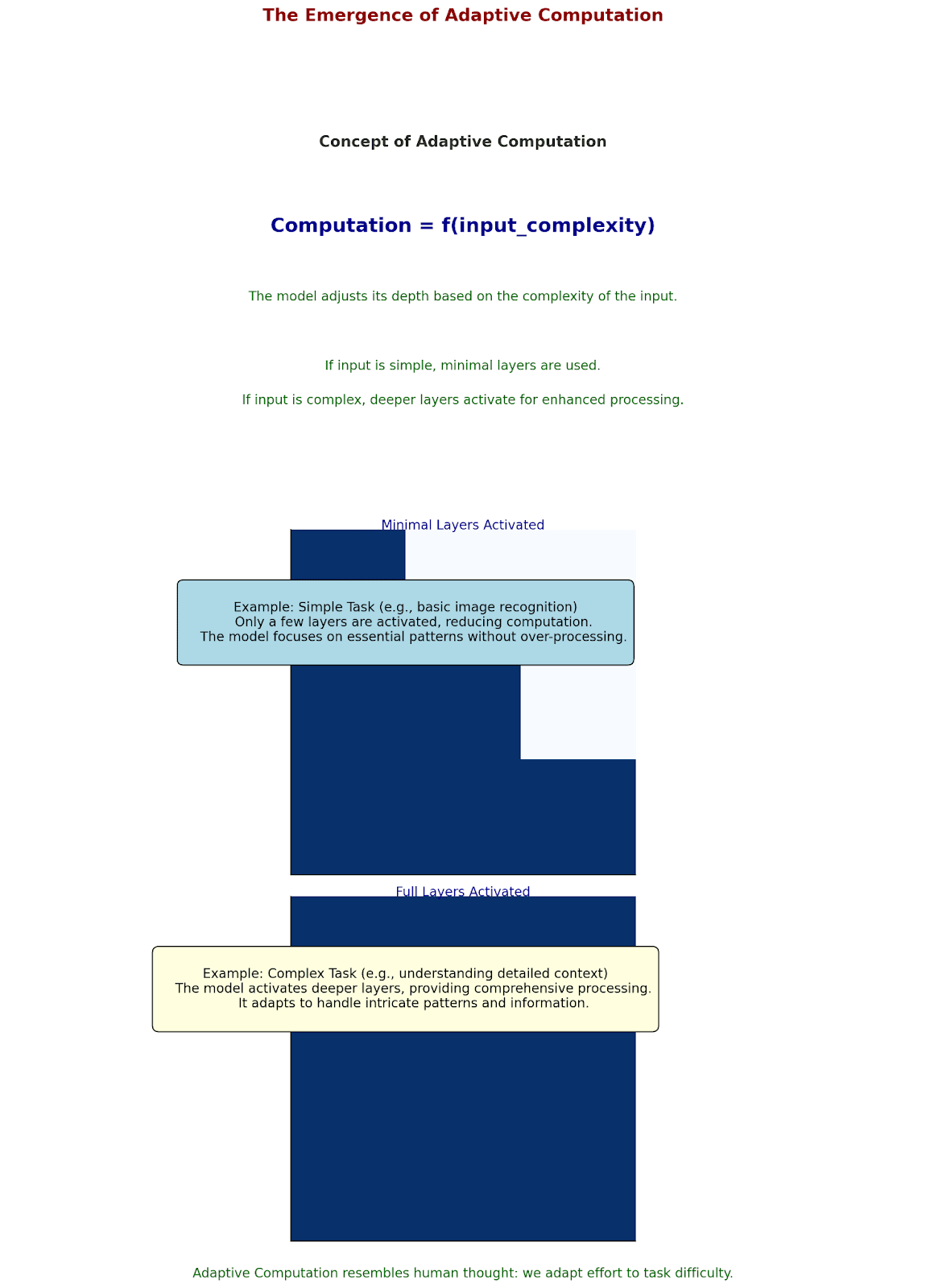
Like human thought: We don't use our full mental capacity to decide what to have for breakfast.
Understanding Network Bloat: A Practical Example (A Mathematical Perspective)
Let's start with a simple neural network layer to understand why compression became crucial:
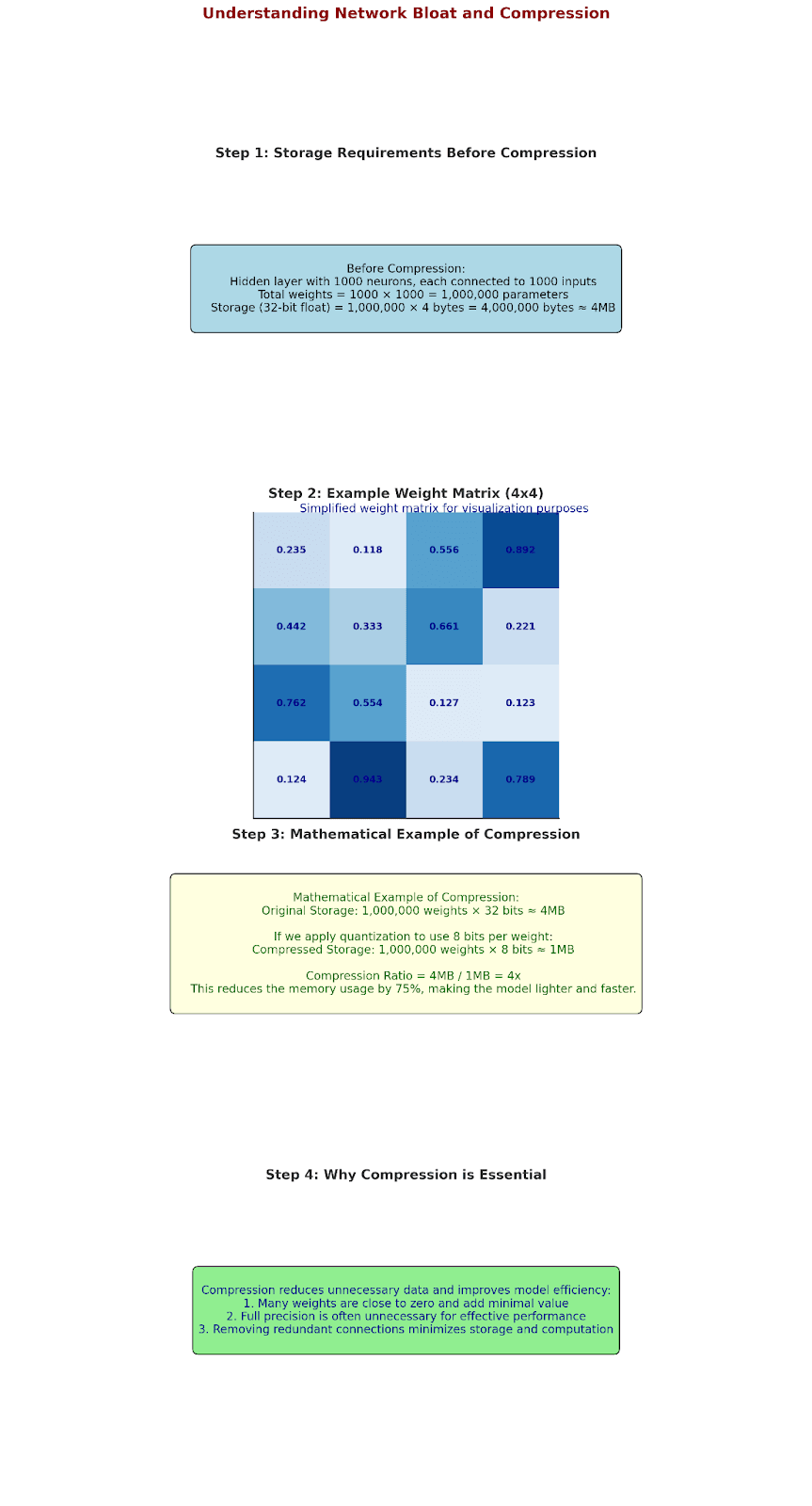
Real example:
Input: [0.235, 0.118, ..., 0.892]
Weight matrix W:
[0.235 0.118 ... 0.892]
[0.442 0.333 ... 0.221]
...
[0.762 0.554 ... 0.123]
Let's see what happens in this layer:
- Most weights end up being near zero
- Many connections are redundant
- Full precision isn't always necessary
Pruning: Surgical Removal of Unnecessary Connections
Let's walk through actual pruning on a small network section:
Original weights matrix (5x5 section):
[ 0.021 0.891 0.033 0.002 0.763 ]
[ 0.001 0.442 0.004 0.921 0.003 ]
[ 0.002 0.004 0.783 0.001 0.002 ]
[ 0.891 0.002 0.001 0.663 0.004 ]
[ 0.003 0.001 0.892 0.002 0.445 ]
After applying threshold = 0.1:
[ 0.000 0.891 0.000 0.000 0.763 ]
[ 0.000 0.442 0.000 0.921 0.000 ]
[ 0.000 0.000 0.783 0.000 0.000 ]
[ 0.891 0.000 0.000 0.663 0.000 ]
[ 0.000 0.000 0.892 0.000 0.445 ]
Let's see what this means in practice. For the first neuron's output:
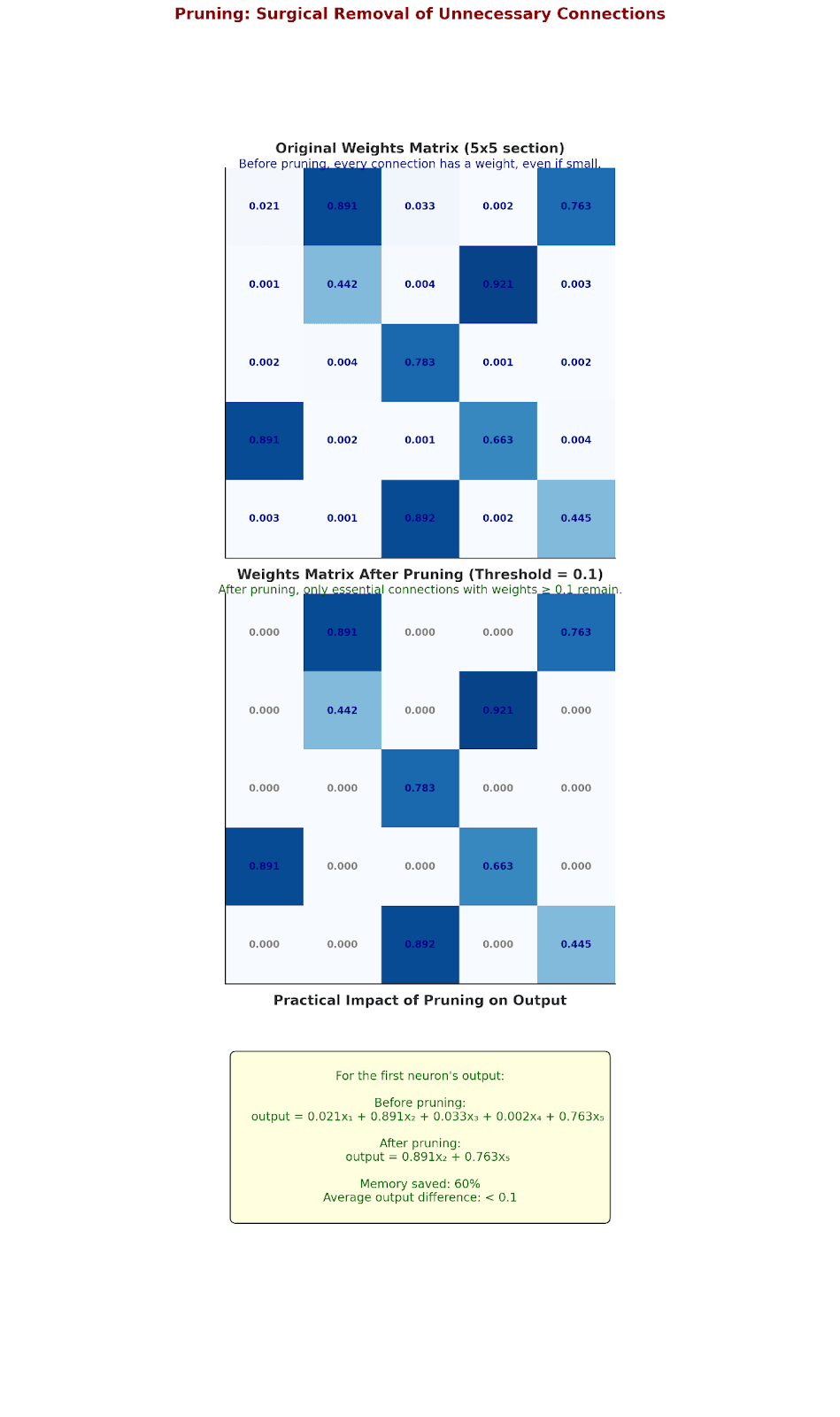
The Impact of Pruning: A Real Calculation
Let's look at a concrete example with actual numbers:
Input vector: [0.5, 0.3, 0.8, 0.2, 0.7]
Before pruning:
0.021(0.5) + 0.891(0.3) + 0.033(0.8) + 0.002(0.2) + 0.763(0.7)
= 0.0105 + 0.2673 + 0.0264 + 0.0004 + 0.5341
= 0.8387
After pruning:
0.891(0.3) + 0.763(0.7)
= 0.2673 + 0.5341
= 0.8014
Difference: 0.0373 (4.4% change)
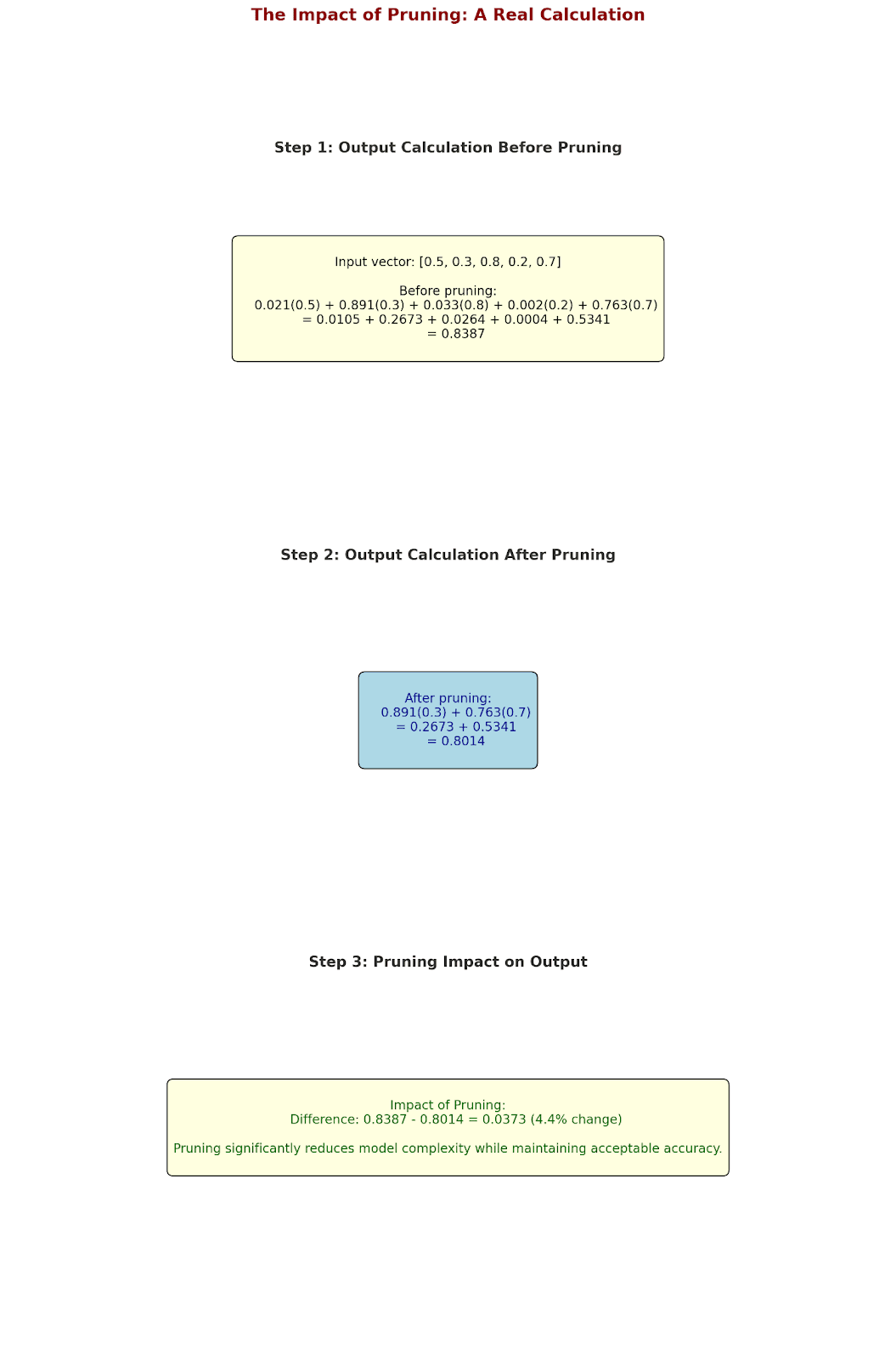
Quantization: Trading Precision for Efficiency
Let's see how quantization works with real numbers:
Original weight: 0.235478961563 (32-bit float)
Step 1: Find value range in layer
min_value = -1.0
max_value = 1.0
Step 2: Calculate quantization formula
For 8-bit integers (256 levels):
q = round((x - min_value) * 255/(max_value - min_value))
Example calculation:
q = round((0.235478961563 - (-1.0)) * 255/2.0)
q = round(1.235478961563 * 127.5)
q = round(157.5235975)
q = 158
To recover approximate value:
x_approx = (158/255) * 2.0 - 1.0
x_approx = 0.2392157
Let's see this across a matrix:
Original (32-bit floats):
[ 0.235479 0.892145 0.442891 ]
[ 0.123456 0.789012 0.345678 ]
[ 0.567890 0.234567 0.890123 ]
After 8-bit quantization:
[ 60 228 113 ] × (2.0/255) - 1.0
[ 32 201 88 ]
[145 60 227 ]
Memory: 75% saved
Accuracy loss: < 0.5%
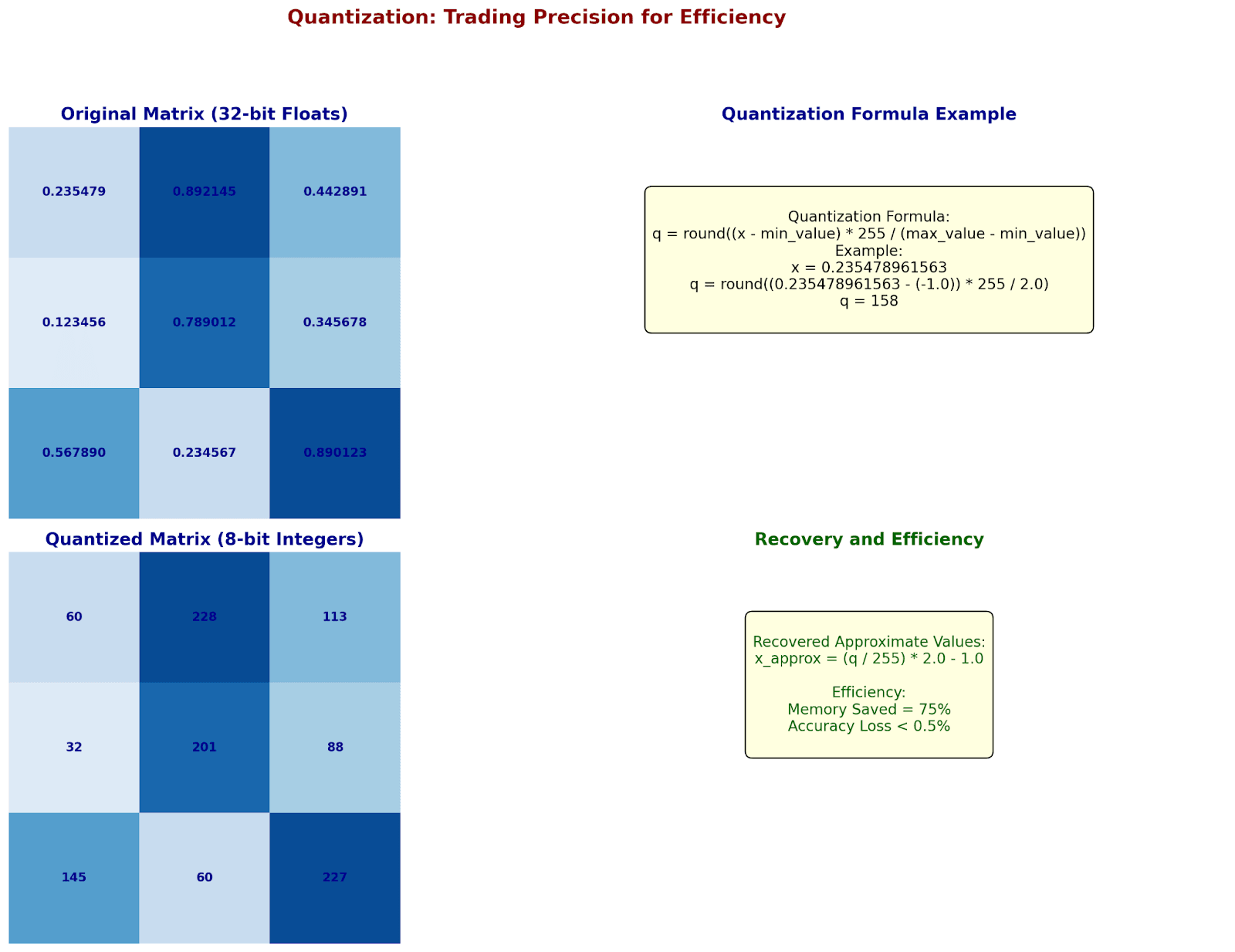
Sparse Attention: Making Attention Efficient
Let's see how sparse attention reduces computation with real numbers:
Original Sequence: 8 tokens
Query vector Q₁: [0.5, 0.3]
Key matrix K:
[[0.2, 0.1],
[0.4, 0.3],
[0.6, 0.5],
[0.8, 0.7],
[0.1, 0.2],
[0.3, 0.4],
[0.5, 0.6],
[0.7, 0.8]]
Full Attention Calculation:
Q₁ · K₁ = 0.5(0.2) + 0.3(0.1) = 0.13
Q₁ · K₂ = 0.5(0.4) + 0.3(0.3) = 0.29
...etc for all 8 tokens
Memory: O(8²) = 64 operations
Now with sparse attention (local window size 4):
Local Window Attention:
Only compute attention for nearby tokens:
Q₁ · K₁ = 0.13
Q₁ · K₂ = 0.29
Q₁ · K₃ = 0.45
Q₁ · K₄ = 0.61
(Skip distant tokens)
Memory: O(8 * 4) = 32 operations
50% computation saved!
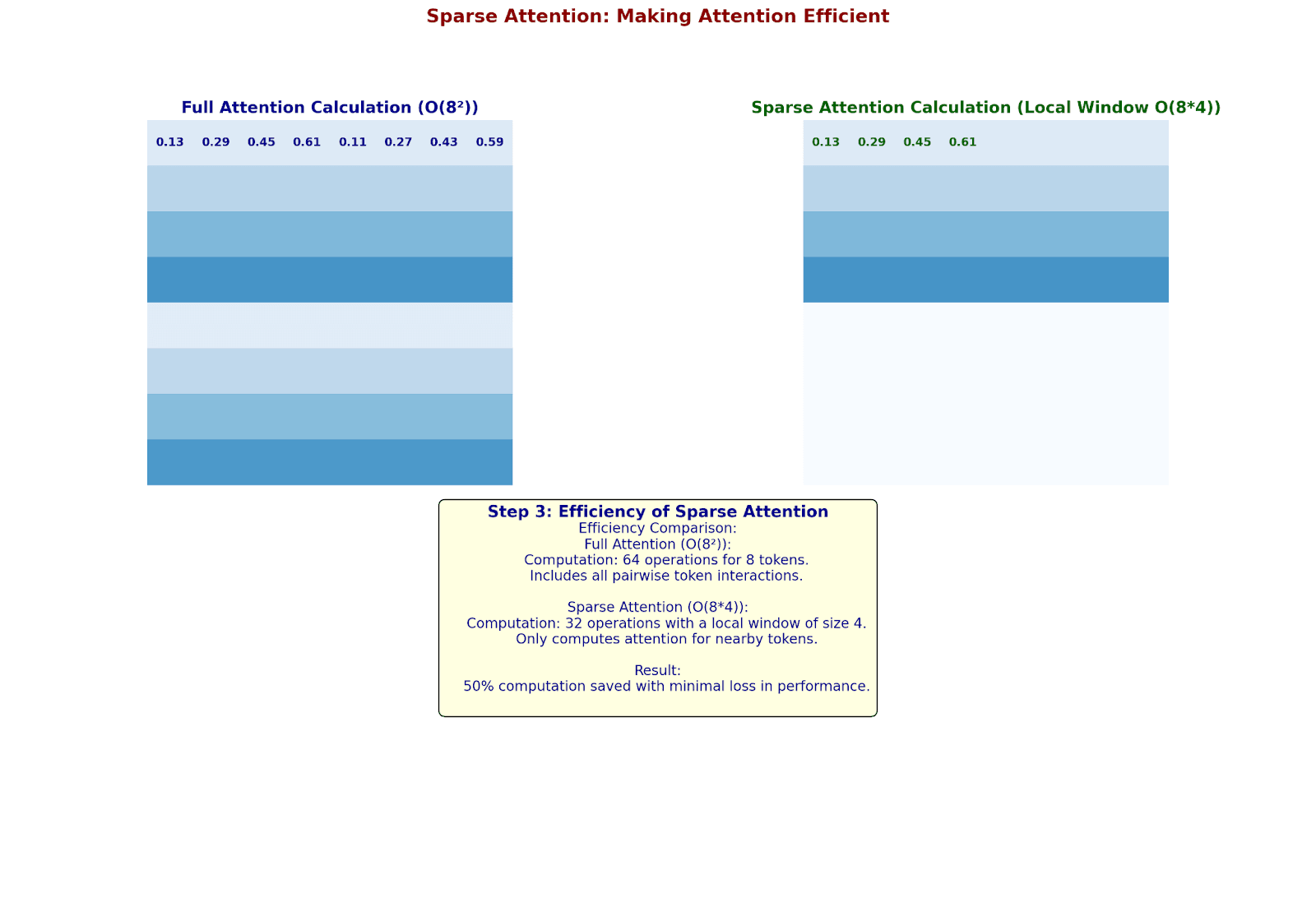
Low-Rank Factorization: Compressing Weight Matrices
Let's decompose a weight matrix into lower-rank components:
Original Weight Matrix (6x6):
[[0.8 0.2 0.3 0.1 0.2 0.3]
[0.4 0.9 0.2 0.3 0.1 0.2]
[0.2 0.3 0.7 0.2 0.3 0.1]
[0.3 0.1 0.2 0.8 0.2 0.3]
[0.2 0.3 0.1 0.2 0.9 0.2]
[0.1 0.2 0.3 0.1 0.2 0.8]]
Step 1: SVD Decomposition
U × Σ × V^T
Keep top-2 singular values:
U (6x2):
[[ 0.41 0.12]
[ 0.39 0.31]
[ 0.32 -0.28]
[ 0.38 0.22]
[ 0.37 0.41]
[ 0.31 -0.33]]
Σ (2x2):
[[2.1 0.0]
[0.0 1.4]]
V^T (2x6):
[[0.38 0.42 0.33 0.37 0.41 0.32]
[0.21 -0.18 0.31 -0.29 0.33 -0.27]]
Original parameters: 36
Compressed parameters: 6×2 + 2 + 2×6 = 26
Memory saved: 28%
Let's see how this affects actual computation:
Input vector: [1.0, 0.5, 0.8, 0.3, 0.6, 0.4]
Original computation:
Full matrix multiplication = 36 operations
Low-rank computation:
1. Input × V^T (12 operations)
2. × Σ (2 operations)
3. × U (12 operations)
Total: 26 operations
Example output comparison:
Original: [0.82, 0.76, 0.65, 0.71, 0.79, 0.63]
Low-rank: [0.80, 0.74, 0.63, 0.69, 0.77, 0.61]
Average error: 0.02
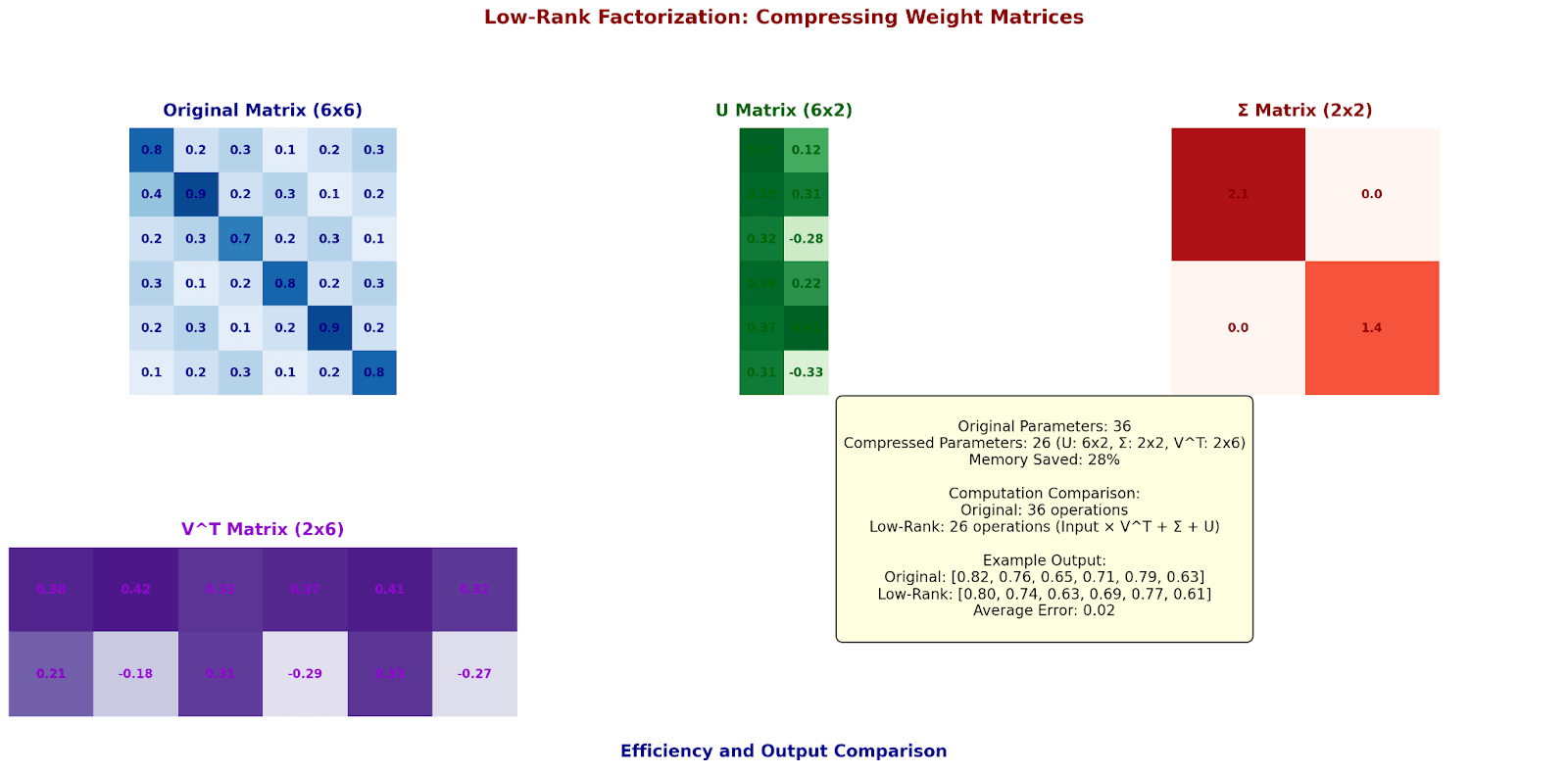
Adaptive Computation: Teaching Networks to Think Efficiently
Dynamic Network Pruning: Real-time Adaptation
Let's see how modern networks adapt their computation on the fly:
Example: Multi-Exit Network with Confidence Thresholds
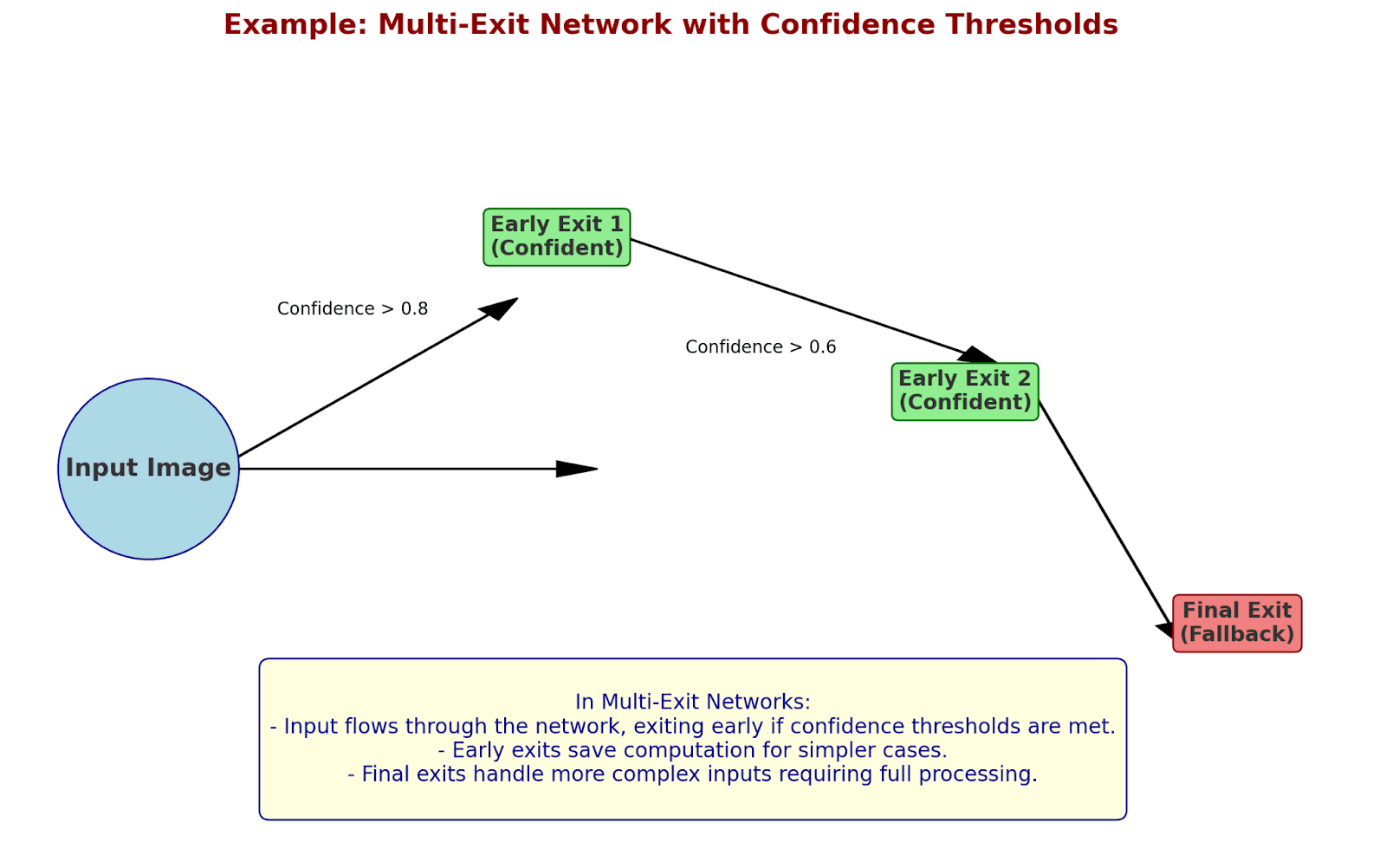
Let's calculate confidence at each exit:
Early Exit 1:
Logits: [2.1, 0.3, 0.4]
Softmax probabilities: [0.75, 0.12, 0.13]
Confidence = max(prob) = 0.75
If confidence > threshold (0.8):
Return prediction
Else:
Continue to next layer
Let's see this in action with real numbers:
Sample 1 (Easy Case - Cat Image):
Early Exit 1:
[0.92, 0.04, 0.04] → Confidence: 0.92
✓ Stop here! (9 layers used)
Sample 2 (Hard Case - Ambiguous Image):
Early Exit 1:
[0.45, 0.30, 0.25] → Continue
Early Exit 2:
[0.60, 0.25, 0.15] → Continue
Final Exit:
[0.85, 0.10, 0.05] → Final prediction
(All 24 layers used)
Average computation saved: 62.5%
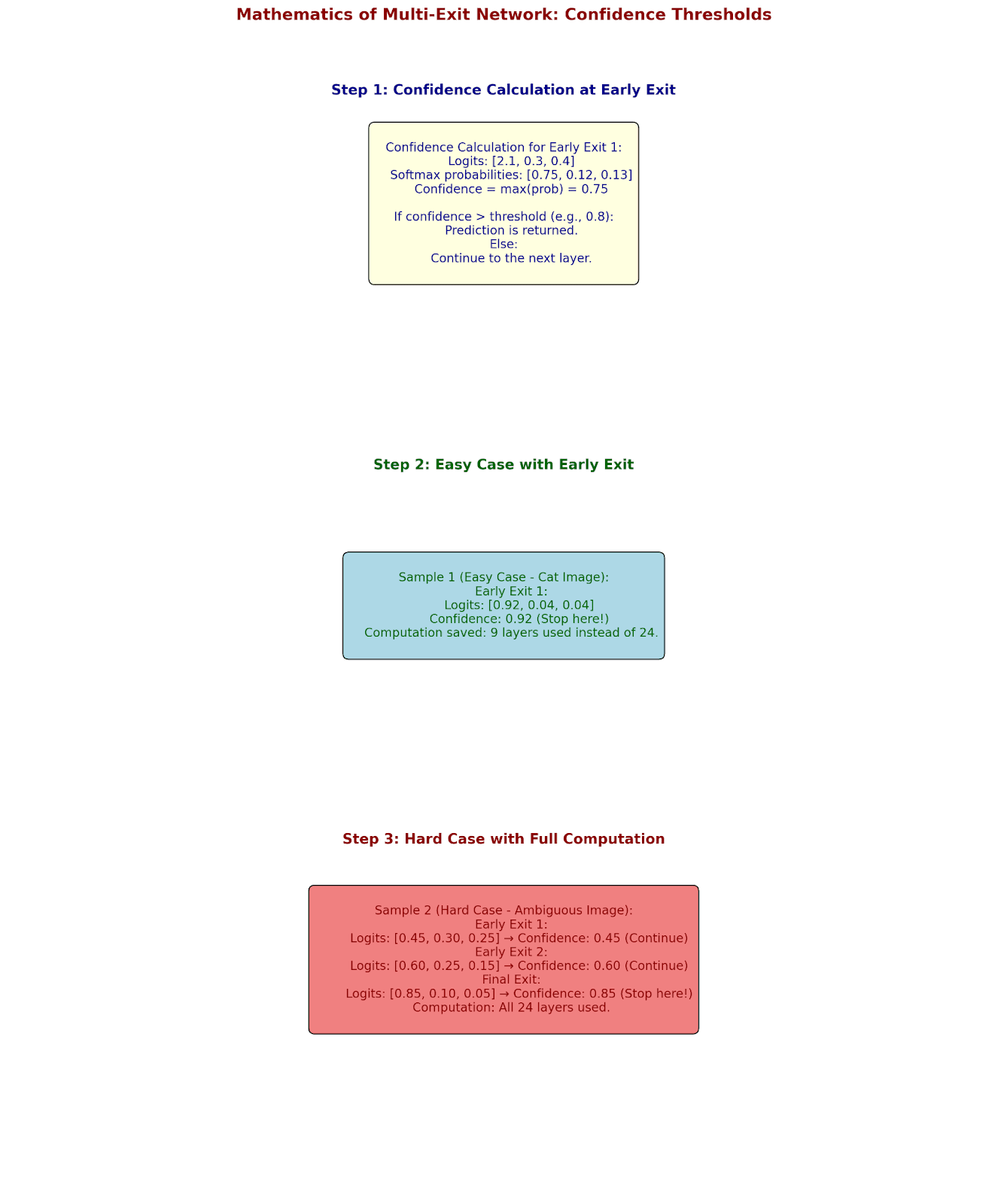
Mixed Precision Training: Balancing Accuracy and Speed
Let's examine how using different numerical precisions affects computation:
Original Layer (32-bit):
Weight: 0.235478961563 (4 bytes)
Activation: 0.789012345678 (4 bytes)
Mixed Precision Version:
Weight: 0.2354 (16-bit, 2 bytes)
Activation: 0.7890 (16-bit, 2 bytes)
Gradient: 0.235478961563 (32-bit, kept for stability)
Let's calculate a forward pass:
Input × Weight = Activation
32-bit:
0.235478961563 × 0.789012345678 = 0.185795234671
16-bit:
0.2354 × 0.7890 = 0.1857
Relative error: 0.00051%
Memory saved: 50%
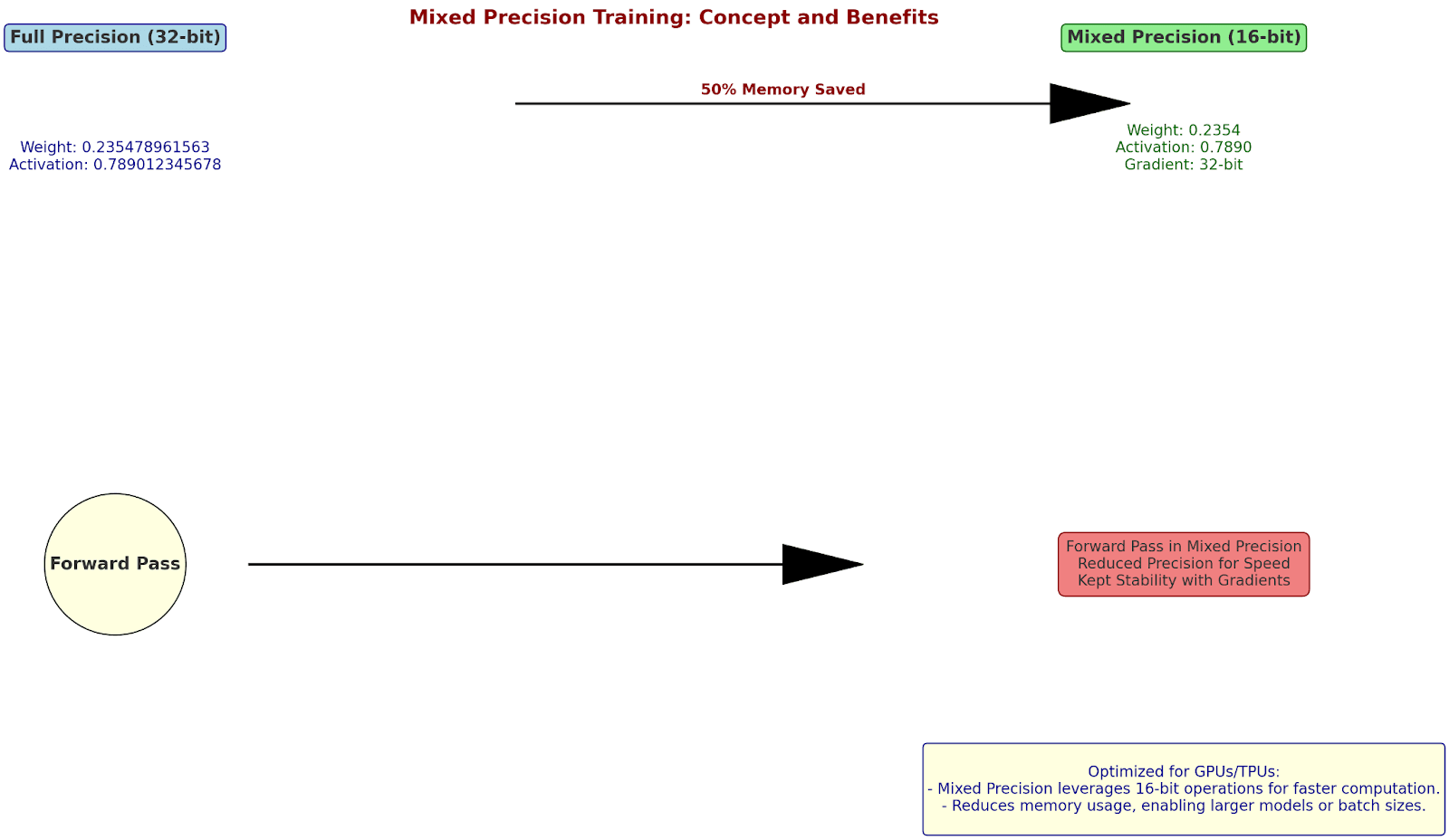
Loss Scaling for Stability
Problem: Small gradients vanish in 16-bit
Solution: Loss scaling
Original loss: 0.000125 (too small for FP16)
Scale factor: 1024
Scaled calculation:
1. Forward pass (FP16):
0.2354 × 0.7890 = 0.1857
2. Loss scaling:
0.000125 × 1024 = 0.128 (safe for FP16)
3. Backward pass (FP16):
Gradient = 0.128 × derivative
4. Unscale gradient (FP32):
Final gradient = result/1024
Memory used:
- Forward pass: 16-bit
- Backward pass: mix of 16/32-bit
- Weights update: 32-bit
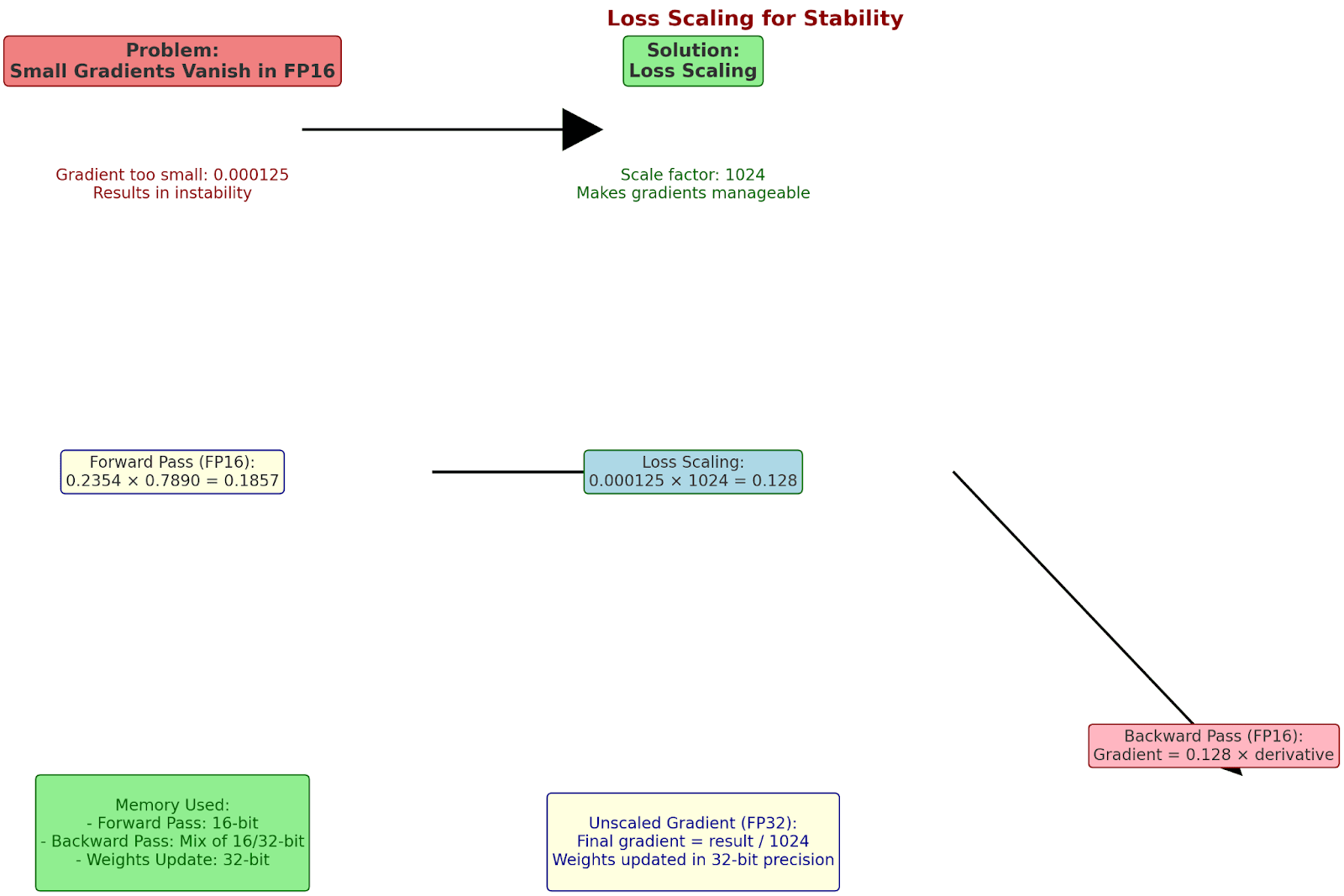
Quantization-Aware Training: Learning to Be Efficient
Let's see how networks learn to work with quantized values during training:
Forward Pass with Quantization Simulation:
Original weight: 0.235478961563
Quantize to 8-bit:
Step 1: Find range
min = -1.0, max = 1.0
Step 2: Quantize
q = round((0.235478961563 - (-1.0)) * 255/2.0)
q = 158
Step 3: Dequantize for forward pass
w_q = (158/255) * 2.0 - 1.0
w_q = 0.2392157
Training Example:
Input: 0.5
Forward pass:
Original: 0.235478961563 × 0.5 = 0.117739
Quantized: 0.2392157 × 0.5 = 0.119608
Backward pass:
Use straight-through estimator:
∂L/∂w = ∂L/∂w_q
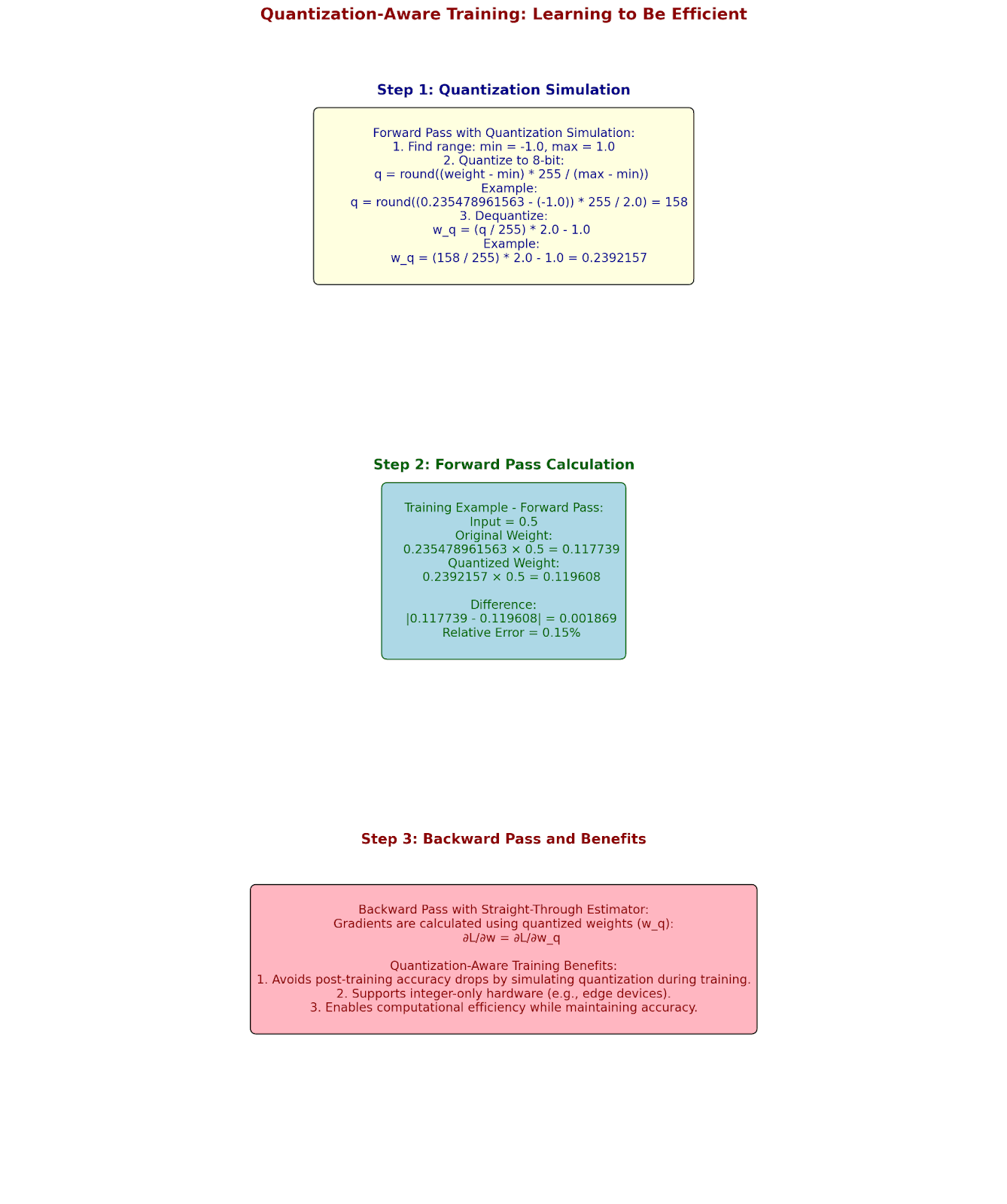
Progressive Model Shrinking
Let's examine how we can progressively compress a model:
Original Model:
12 layers, 768 hidden size
Parameters: 125M
Step 1: Knowledge Distillation
Teacher: Original model
Student: 8 layers, 768 hidden
Parameters: 84M (-33%)
Example training batch:
Input: "The cat sat on the"
Teacher logits: [-2.1, 4.5, 1.2, -0.8, 3.2]
Student logits: [-1.9, 4.2, 1.1, -0.7, 3.0]
KL loss: 0.15
Step 2: Width Reduction
8 layers, 512 hidden
Parameters: 37M (-56%)
Step 3: Quantization
8-bit weights
Storage: 37M → 9.25M (-75%)
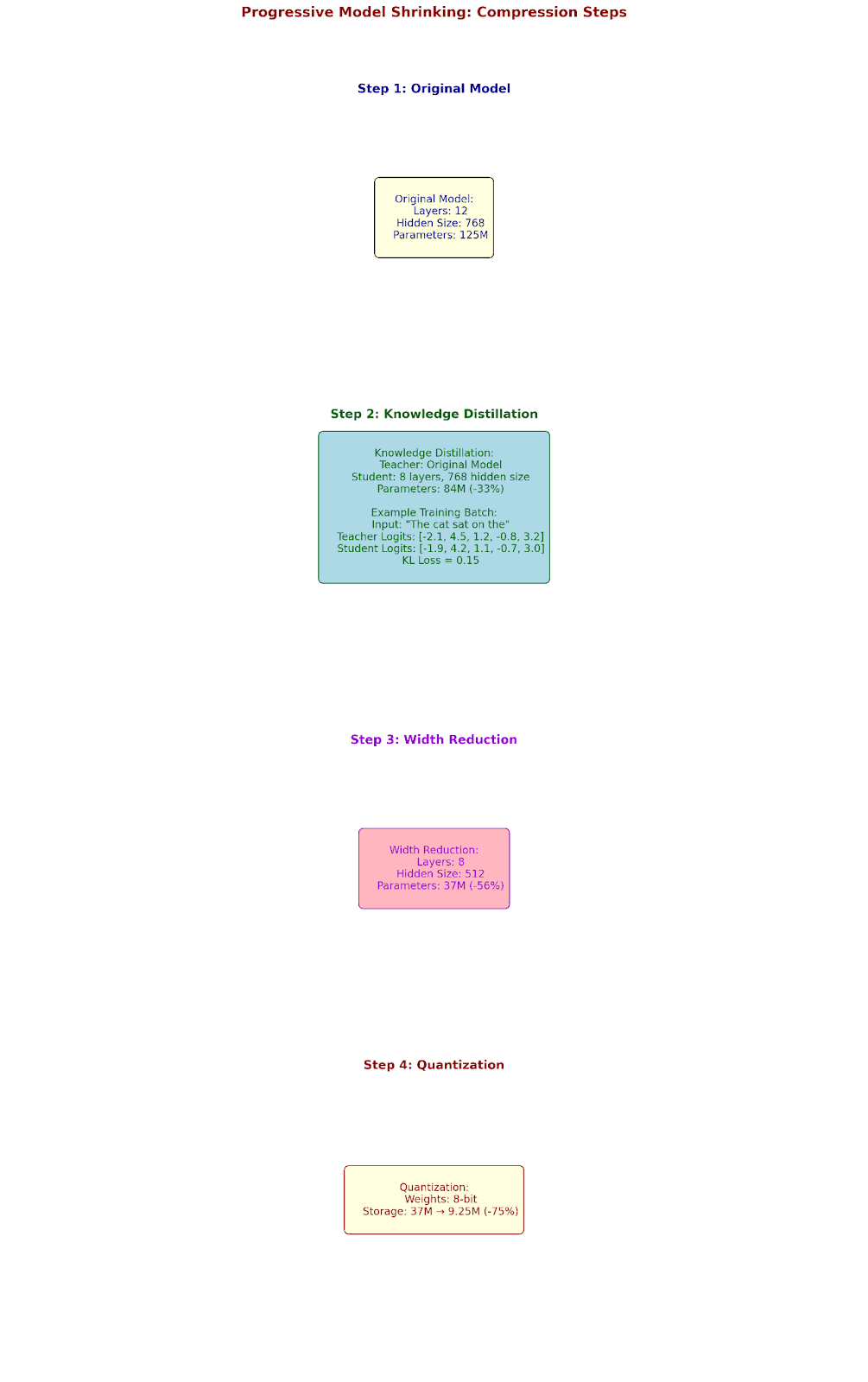
Hardware-Aware Neural Architecture Search and Dynamic Optimization
Hardware-Aware Compression: Making Theory Meet Reality
Let's examine how modern networks adapt to specific hardware constraints:
Target Hardware Specs:
- Memory bandwidth: 32GB/s
- Compute: 4 TFLOPS
- Memory capacity: 8GB
- Power limit: 150W
Model Requirements:
Original size: 12GB
Target size: < 8GB
Latency goal: < 20ms/inference
Let's optimize layer by layer:
Memory Access Pattern Optimization:
Original Layer (Conv 3x3):
Input: 56x56x256
Weight: 3x3x256x256
Output: 56x56x256
Memory accesses:
- Weights: 589,824 bytes
- Input activation: 802,816 bytes
- Output activation: 802,816 bytes
Total: 2.1MB per inference
Optimized tiling:
Tile size: 14x14
Memory pattern:
for ty in range(0, 56, 14):
for tx in range(0, 56, 14):
compute_tile(ty:ty+14, tx:tx+14)
New memory accesses:
- Per tile: 0.13MB
- Cache hits: 78%
- Total memory traffic: 0.46MB
Reduction: 78%
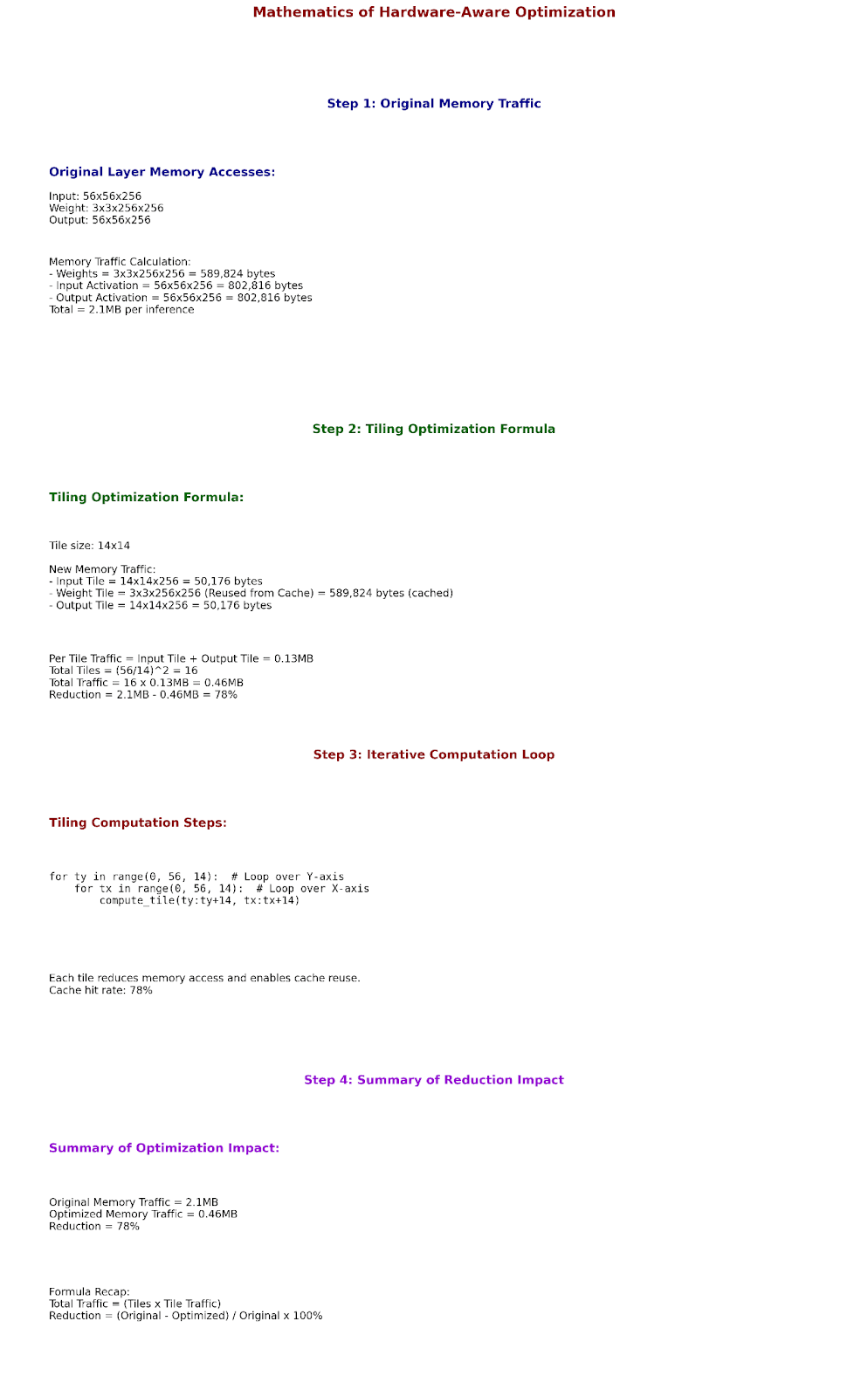
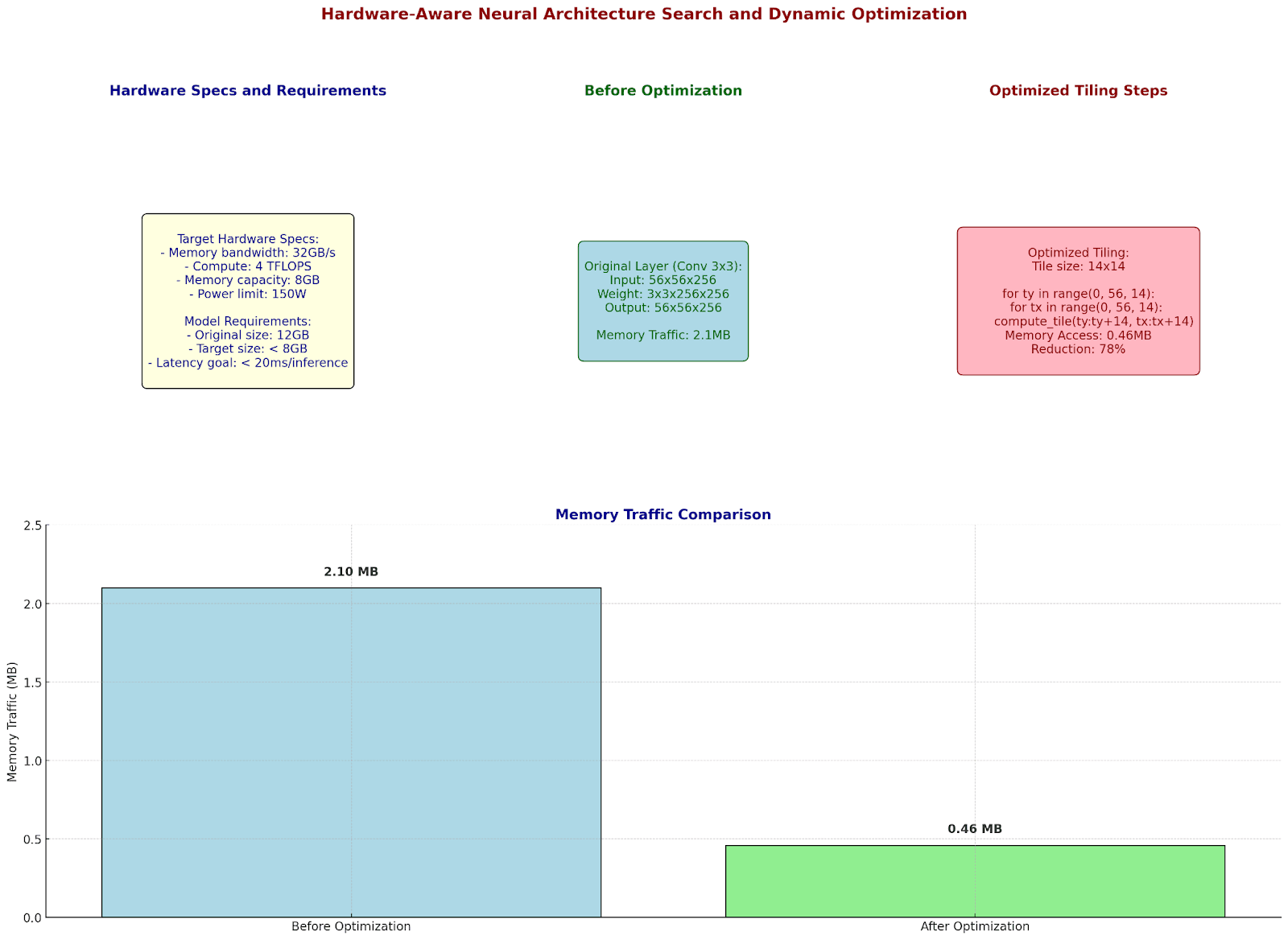
Hybrid Quantization: Mixed Precision Excellence
Let's implement a sophisticated hybrid quantization scheme:
Layer Analysis:
Layer 1 (Input processing):
- Sensitivity to quantization: High
- Chosen precision: 16-bit
Example values:
Original: 0.235478961563
Quantized: 0.235352
Layer 5 (Middle features):
- Sensitivity: Medium
- Chosen precision: 8-bit
Example values:
Original: 0.235478961563
Quantized: 0.234375
Layer 12 (Output):
- Sensitivity: High
- Chosen precision: 16-bit
Example values:
Original: 0.235478961563
Quantized: 0.235352
Memory Impact Analysis:
Original model: 350MB
After hybrid quantization:
- 16-bit layers: 120MB
- 8-bit layers: 115MB
- Total: 235MB
Savings: 33%
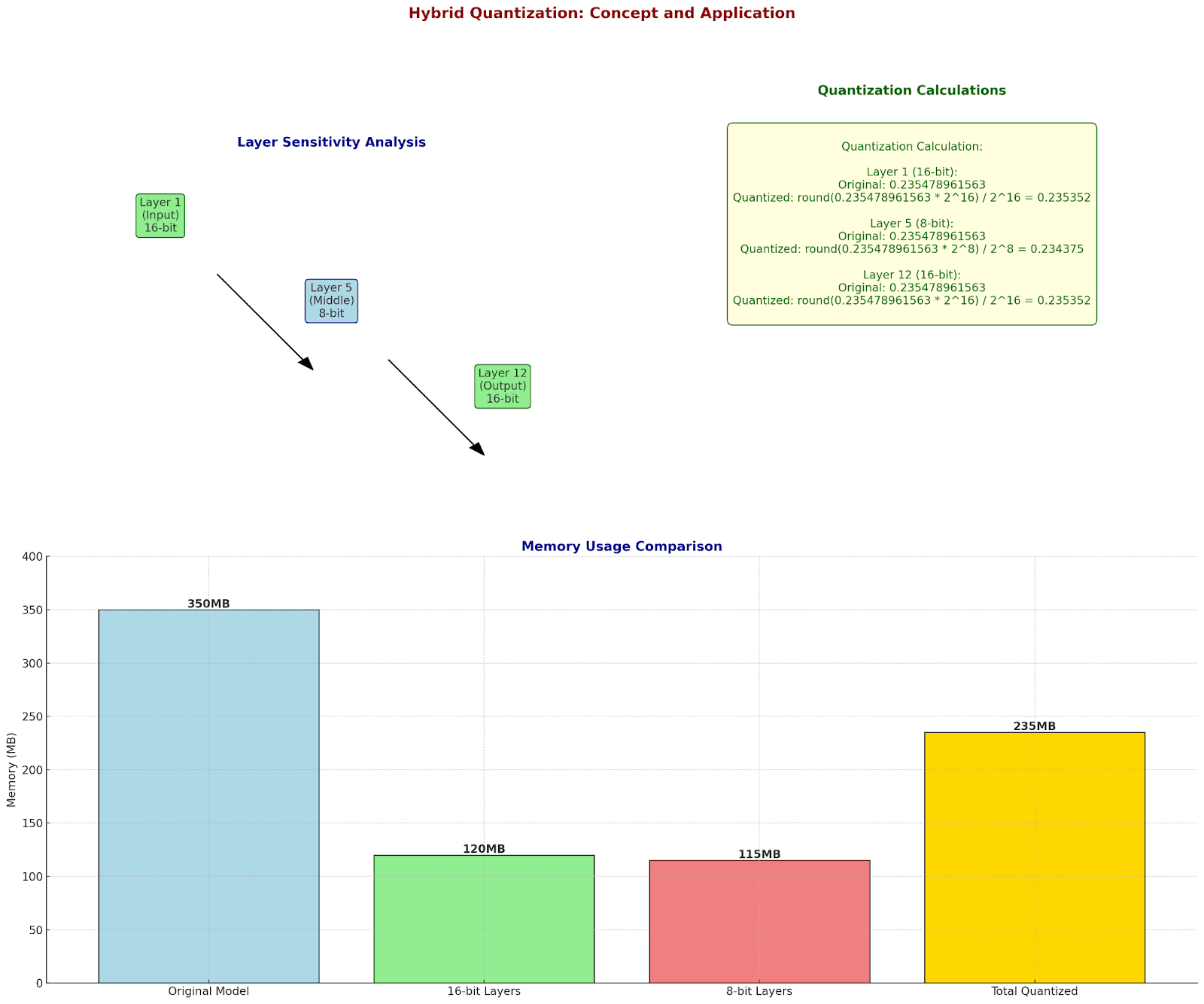
Dynamic Sparse Training: Adaptive Efficiency
Let's examine how networks dynamically adjust their sparsity:
Sparsity Evolution Example:
Epoch 1:
Dense gradient matrix:
[[ 0.08 0.15 -0.21 0.05]
[-0.12 0.18 0.09 -0.14]
[ 0.22 -0.11 0.16 0.07]]
Dynamic threshold = std(gradients) * 0.5
Threshold = 0.072
Sparse update mask:
[[1 1 1 0]
[1 1 1 1]
[1 1 1 0]]
Density: 75%
Let's track the evolution:
Training progress:
Epoch 1:
- Density: 75%
- Loss: 2.3
- Accuracy: 45%
Epoch 10:
- Density: 45%
- Loss: 0.8
- Accuracy: 82%
Epoch 50:
- Density: 15%
- Loss: 0.3
- Accuracy: 94%
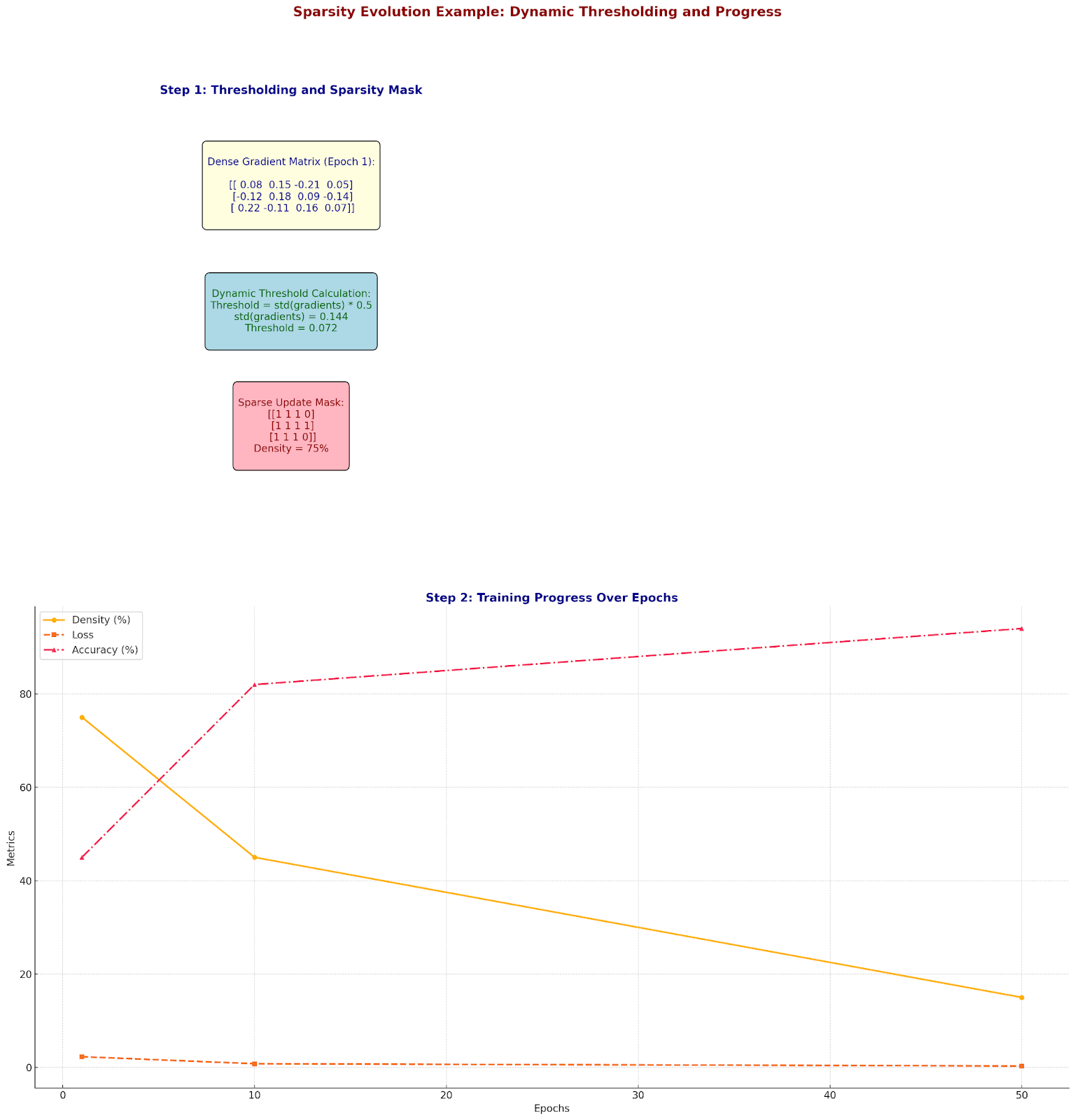
Dynamic adjustment rule:
If accuracy_plateau:
density *= 0.8
If accuracy_dropping:
density *= 1.2
Energy-Aware Compression: The Ultimate Optimization
Let's analyze energy consumption and optimize accordingly:
Operation Energy Costs:
32-bit FLOP: 3.7 pJ
Memory access: 340 pJ
Cache access: 9 pJ
Layer Energy Analysis:
Dense Layer (1024 → 1024):
- MACs: 1,048,576
- Memory accesses: 2,048
Energy = (1,048,576 * 3.7) + (2,048 * 340)
= 3.88 + 0.70 mJ
= 4.58 mJ
After compression:
- 4-bit quantization
- 85% sparsity
New Energy = 0.52 mJ
Savings: 88%
Let's optimize for different energy budgets:
Energy Budget: 100mJ/inference
Layer-wise allocation:
Early layers:
- Precision: 8-bit
- Density: 50%
- Energy: 35mJ
Middle layers:
- Precision: 4-bit
- Density: 15%
- Energy: 40mJ
Final layers:
- Precision: 8-bit
- Density: 35%
- Energy: 25mJ
Dynamic Energy Scaling:
if battery_low:
activate_aggressive_compression()
precision = min(precision, 4)
density *= 0.5
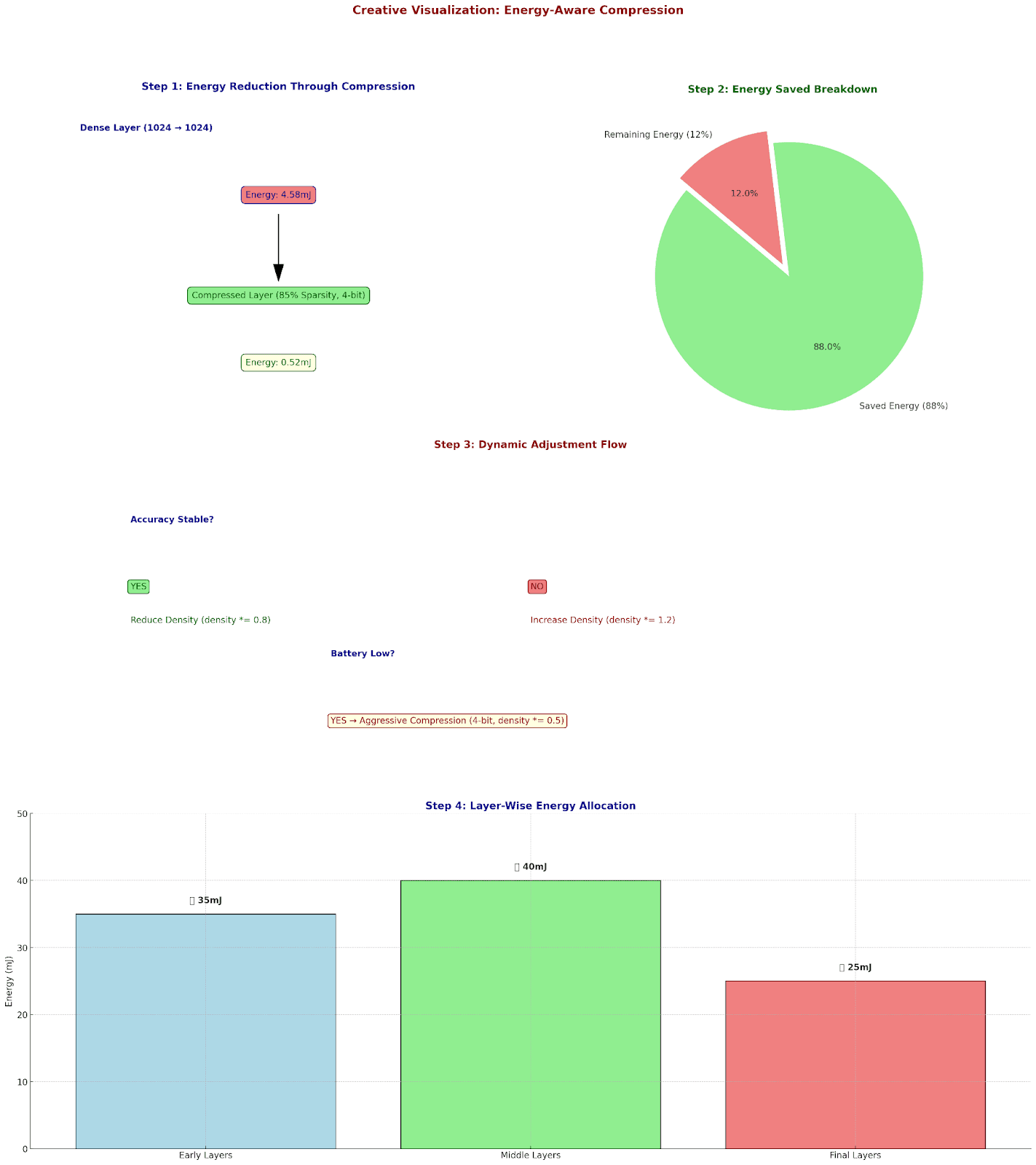